Artificial Intelligence (AI) has become an integral part of our lives, from personalized recommendations on streaming platforms to voice assistants that can answer our questions in seconds. However, as AI continues to evolve and become more complex, concerns about its transparency and trustworthiness have emerged. Enter explainability โ the key ingredient that fuels trust in AI models. In this article, we will delve into the concept of explainability, its impact on trust, and its role in the future of AI.
โ
Understanding the Concept of Explainability in AI
โ
Explainability refers to the ability to understand and interpret the decisions made by AI models. It is the process of uncovering how and why certain predictions or recommendations are made. The importance of transparency in AI models cannot be overstated. Users want to know why an AI system suggested a certain course of action or reached a particular conclusion. When AI models are explainable, users can trust that the decisions made are rational, fair, and unbiased.
โ
The Importance of Transparency in AI Models
โ
Transparency lays the foundation for trust in AI models. When users have visibility into the inner workings of an AI system, they can understand the factors considered and the logic behind the decisions. This transparency fosters trust, as users can evaluate the system's reasoning and judge its reliability.
โ
Transparency in AI models also enables users to identify potential biases or discriminatory patterns. By understanding how the AI system arrived at its decisions, users can assess whether the model is treating different groups equitably. This is particularly crucial in sensitive domains such as hiring, lending, or criminal justice, where biased decisions can have profound consequences.
โ
Moreover, transparency allows users to provide feedback and suggest improvements to the AI system. When users can comprehend the decision-making process, they can offer valuable insights and help refine the model's performance. This collaborative approach enhances the overall effectiveness and accuracy of AI systems.
โ
Defining Explainability in the Context of AI
โ
In the context of AI, explainability entails the ability to provide clear explanations for the results generated by AI models. It involves demystifying the black box nature of AI algorithms and making their decision-making process understandable to humans. By doing so, AI models can be held accountable and their decisions can be justified.
โ
Explainability in AI is not a one-size-fits-all concept. Different stakeholders may require different levels of explanation depending on their roles and responsibilities. For example, regulators may need a detailed understanding of the model's inner workings to ensure compliance with legal and ethical standards. On the other hand, end-users may only need a high-level overview to make informed decisions based on the AI system's recommendations.
โ
There are various techniques and approaches to achieve explainability in AI models. These include rule-based systems, model-agnostic methods, and interpretable machine learning algorithms. Each approach has its strengths and limitations, and the choice of method depends on the specific requirements and constraints of the AI application.
โ
Explainability is an ongoing area of research and development in the field of AI. As AI models become more complex and sophisticated, ensuring their explainability becomes increasingly challenging. However, the efforts to enhance transparency and accountability in AI are crucial for building trust and fostering responsible AI adoption in various domains.
โ
โ

โ
โ
The Link Between Explainability and Trust
โ
Explainability and trust in AI models are intricately connected. When users can understand how AI models reach their conclusions, they feel more confident in the system's reliability. Explainability also helps to mitigate user concerns about potential biases or hidden agendas within the AI models.
โ
Understanding the inner workings of AI models is crucial for users to have faith in the technology. The ability to explain the decision-making process behind AI outputs provides transparency and accountability. It allows users to assess the validity of the results and ensures that the AI system is not making arbitrary or biased decisions.
โ
Moreover, explainability plays a significant role in building trust. It goes beyond just providing an explanation; it involves presenting the information in a way that users can comprehend easily. By providing a clear and interpretable explanation for AI outputs, users can follow the logic and reasoning behind the system's decisions.
โ
Building Trust in AI Through Explainability
โ
Explainability plays a crucial role in building trust. By providing a clear and interpretable explanation for AI outputs, users can assess the validity of the results. This fosters a sense of trust and confidence in the system, leading to greater user satisfaction and acceptance of AI technology.
โ
Imagine a scenario where a healthcare AI system recommends a specific treatment plan for a patient. Without explainability, the patient and the healthcare provider might be hesitant to follow the recommendation blindly. However, if the AI system can provide a detailed explanation of how it arrived at the treatment plan, including the relevant medical literature and patient-specific data, it instills confidence in the decision-making process.
โ
Furthermore, explainability helps users understand the limitations and potential risks associated with AI models. It allows them to make informed decisions and be aware of any biases or uncertainties in the system's predictions. This knowledge empowers users to critically evaluate the AI technology and trust it when appropriate.
โ
โ
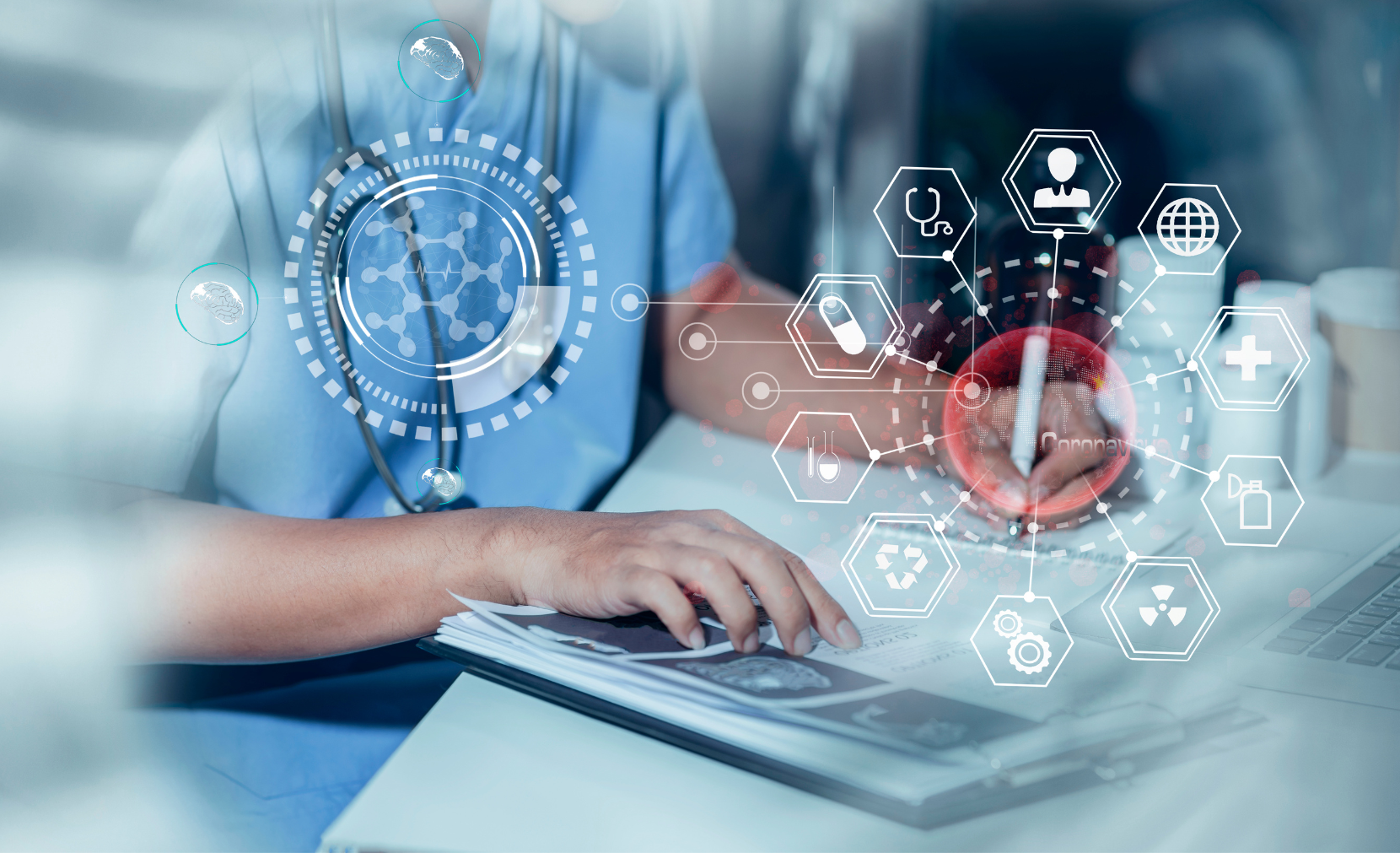
โ
โ
The Role of Explainability in User Trust
โ
User trust is indispensable in the success of AI adoption. Explainability addresses the fundamental human need for understanding. When users can follow the decision-making process of AI models, they are more likely to trust the system and rely on its recommendations or predictions. This trust factor is essential, especially in sensitive domains such as healthcare and finance.
โ
In healthcare, for instance, patients and healthcare providers need to have confidence in AI systems that assist in diagnosing diseases or suggesting treatment plans. By providing explainability, AI models can demonstrate their reliability and help users understand the reasoning behind their recommendations. This understanding fosters trust, leading to better collaboration between AI systems and human experts.
โ
Similarly, in the financial sector, explainability is crucial for users to trust AI models that make investment recommendations or assess creditworthiness. By explaining the factors and variables considered in the decision-making process, AI models can provide transparency and accountability. This transparency helps users evaluate the fairness and accuracy of the AI system's predictions, ultimately building trust in its capabilities.
โ
The Impact of Explainability on AI Adoption
โ
The adoption of AI technology faces several challenges, including concerns about algorithmic biases, lack of transparency, and the fear of job displacement. Explainability can act as a game-changer in overcoming these barriers and accelerating AI adoption.
โ
Overcoming AI Adoption Challenges with Explainability
โ
Explainability can address concerns around algorithmic bias by exposing any biases present in the training data or model design. By understanding the decision-making process, users can identify and correct potential biases, improving the fairness and equity of AI systems. This not only promotes trust but also makes AI more inclusive and accessible to all.
โ
How Explainability Enhances Transparency
โ
Transparency is a critical factor in AI adoption. Without understanding how AI systems arrive at their decisions, users may be hesitant to trust and rely on them. Explainability provides a window into the inner workings of AI models, allowing users to comprehend the factors and variables that influence the decision-making process. This transparency fosters confidence and enables users to make informed decisions based on AI-generated insights.
โ
Moreover, explainability helps in identifying and rectifying errors or biases that may occur during the training or implementation of AI models. By providing a clear and understandable explanation of the decision-making process, users can detect any inconsistencies or unintended consequences that may arise. This enables them to take corrective measures and improve the accuracy and reliability of AI systems.
โ
Addressing the Fear of Job Displacement
โ
One of the major concerns surrounding AI adoption is the fear of job displacement. Many individuals worry that AI technologies will replace human workers, leading to unemployment and economic instability. However, explainability can help alleviate these fears by showcasing the collaborative nature of AI-human interaction.
โ
Explainability highlights how AI systems can complement and enhance human capabilities rather than replace them entirely. By providing insights into the decision-making process, explainable AI empowers users to understand the rationale behind AI-generated recommendations or predictions. This allows humans to leverage AI technology as a tool to augment their own skills and expertise, leading to increased productivity and job satisfaction.
โ
Furthermore, explainability enables users to actively participate in the development and improvement of AI systems. By understanding how AI models work, users can provide feedback, suggest improvements, and contribute to the ongoing refinement of AI technologies. This collaborative approach not only ensures that AI systems align with human values and needs but also creates opportunities for skill development and job creation in AI-related fields.
โ
โ

โ
โ
Conclusion
โ
Explainability plays a crucial role in overcoming the challenges associated with AI adoption. By enhancing transparency, addressing algorithmic biases, and alleviating the fear of job displacement, explainable AI promotes trust, inclusivity, and collaboration. As AI continues to advance, the integration of explainability will be essential in building a future where AI technologies are widely adopted and beneficial to society.