Advancements in artificial intelligence (AI) have revolutionized various aspects of our lives. From personalized recommendations to healthcare diagnostics, AI systems have become an integral part of decision-making processes. However, concerns have been raised regarding the fairness, accountability, and transparency of these AI systems. It is crucial to address these concerns to ensure that AI technology works in the best interest of all individuals and society as a whole.
โ
Understanding the Importance of Fair, Accountable, and Transparent AI
โ
The first step in building an AI that is fair, accountable, and transparent is to understand the significance of these factors. Fairness in AI refers to the equitable treatment of all individuals, regardless of their race, gender, or socioeconomic background. It aims to mitigate biases and ensure equal opportunities for everyone.
โ
For example, imagine an AI system used in the hiring process. If the system is not designed to be fair, it may inadvertently discriminate against certain candidates based on their demographic information. This could lead to a biased selection process, where qualified candidates are overlooked simply because of their background. By prioritizing fairness in AI, we can create systems that promote diversity and inclusion, allowing for a more equitable society.
โ
Accountability in AI systems entails identifying individuals or entities responsible for the decisions made by AI algorithms. This involves establishing guidelines and frameworks to allocate responsibility and hold individuals accountable for any negative outcomes resulting from AI decisions.
โ
Consider an AI system used in autonomous vehicles. If the system makes a decision that leads to an accident, it is crucial to determine who is responsible for that decision. Is it the developer who wrote the algorithm, the company that deployed the system, or the user who was supposed to be supervising the vehicle? By establishing clear lines of accountability, we can ensure that AI systems are developed and used responsibly, minimizing the potential for harm.
โ
Transparency is a key factor in building trustworthy AI systems. It involves making the decision-making process of AI algorithms understandable and explainable to users. Transparent AI helps build trust and enables users to have a better understanding of how and why certain decisions are made.
โ
Take, for instance, an AI system used in healthcare to diagnose diseases. If the system provides a diagnosis without any explanation or justification, it may be difficult for healthcare professionals to trust its recommendations. However, if the system can provide a clear explanation of how it arrived at a particular diagnosis, healthcare professionals can better evaluate its accuracy and make informed decisions. Transparency in AI empowers users to have more confidence in the technology and ensures that its decisions are not seen as arbitrary or untrustworthy.
โ
โ

โ
โ
Steps to Building a Fair AI
โ
Defining Fairness in AI
โ
In order to build a fair AI system, it is crucial to have a clear understanding of what fairness means in the context of AI. Fairness can be defined based on various factors, such as avoiding disparate impact, promoting equal opportunity, or considering individual preferences.
โ
When it comes to avoiding disparate impact, it means ensuring that the AI system does not disproportionately harm or benefit certain groups of people. For example, if an AI system is used in the hiring process, it should not discriminate against candidates based on their gender, race, or other protected characteristics. Promoting equal opportunity means that the AI system should provide equal chances for everyone, regardless of their background or circumstances. Lastly, considering individual preferences means that the AI system should take into account the unique needs and desires of each individual user.
โ
Data Collection and Bias Mitigation
โ
Data collection plays a crucial role in AI development. It is important to ensure that the data used to train AI models is diverse, representative, and free from biases. Biases in the data can lead to biased outcomes and perpetuate discrimination.
โ
One way to mitigate bias in data is to ensure that the data collection process is inclusive and representative of the population that the AI system will interact with. This means collecting data from diverse sources and ensuring that underrepresented groups are not overlooked. Additionally, it is important to regularly review and update the data to account for any changes in society or user preferences.
โ
Bias mitigation techniques can also be applied to the data itself. This involves identifying and removing biased attributes that may influence the AI system's decision-making process. For example, if an AI system is being trained to predict loan approvals, it is important to remove any attributes that are correlated with protected characteristics, such as race or gender, to ensure fair and unbiased outcomes.
โ
Algorithm Selection and Fairness Considerations
โ
When selecting algorithms for AI systems, it is important to consider their potential impact on fairness. Some algorithms may inherently introduce biases, while others may be more suitable for promoting fairness.
โ
One approach to promoting fairness is to use algorithms that have been specifically designed or modified to reduce bias. These algorithms may incorporate fairness constraints or adjustments to ensure that the outcomes are not influenced by protected characteristics. However, it is important to note that fairness is a complex and multidimensional concept, and there may be trade-offs between different fairness criteria.
โ
Thoroughly assessing and evaluating the fairness implications of different algorithms is crucial. This can involve conducting fairness audits, which analyze the impact of the algorithms on different demographic groups. It is also important to involve diverse stakeholders, such as ethicists, social scientists, and representatives from affected communities, in the decision-making process to ensure a comprehensive and inclusive approach to fairness.
โ
Testing AI for Fairness
โ
Regular testing for fairness is essential to ensure that AI systems are not perpetuating biases. Testing should involve evaluating the system's performance across different demographic groups and identifying and addressing any discrepancies or unfair outcomes.
โ
One way to test for fairness is to use fairness metrics, which quantify the extent to which the AI system's outcomes differ across different groups. These metrics can help identify any disparities and guide efforts to mitigate them. Additionally, user feedback and input should be actively sought and considered to ensure that the AI system is meeting the needs and expectations of its users in a fair and equitable manner.
โ
It is important to note that fairness is an ongoing process and requires continuous monitoring and improvement. As societal norms and values evolve, so should the definition and implementation of fairness in AI systems.
โ
โ

โ
โ
Ensuring Accountability in AI Development
โ
Artificial Intelligence (AI) development has the potential to revolutionize various industries and improve efficiency. However, it is crucial to ensure accountability throughout the AI development process to address potential biases, unfair practices, and ethical concerns. This can be achieved through the implementation of clear responsibility guidelines, robust monitoring systems, and regular auditing.
โ
Establishing Clear Responsibility Guidelines
โ
Clear guidelines should be established to define the responsibilities of individuals involved in AI development. This includes outlining the roles and responsibilities of data scientists, algorithm developers, and decision-makers. By establishing clear responsibility guidelines, accountability can be promoted throughout the AI development process.
โ
For example, data scientists should be responsible for collecting and preparing the data used for training AI algorithms. Algorithm developers should ensure that the algorithms are designed to be fair, unbiased, and transparent. Decision-makers should be accountable for the decisions made based on the output of AI systems.
โ
Furthermore, these guidelines should also address potential ethical concerns, such as privacy, security, and the impact of AI systems on society. By clearly defining the responsibilities of each stakeholder, it becomes easier to hold individuals accountable for their actions and decisions.
โ
Implementing Robust Monitoring Systems
โ
Monitoring AI systems is essential for ensuring accountability. Robust monitoring systems can capture and record the decisions made by AI algorithms, allowing for retrospective analysis and identification of any issues or biases. Regular monitoring helps track the performance and impact of AI systems and holds individuals accountable for their decisions.
โ
One way to implement robust monitoring systems is through the use of comprehensive logging. This involves recording all inputs, outputs, and decisions made by AI algorithms. By analyzing these logs, it becomes possible to identify any patterns of bias or unfairness in the decision-making process.
โ
In addition to logging, real-time monitoring can also be employed to detect any anomalies or deviations from expected behavior. This can help identify potential issues before they have a significant impact. By continuously monitoring AI systems, accountability can be ensured throughout their lifecycle.
โ
Regular Auditing for Accountability
โ
Conducting regular audits of AI systems can help identify any biases or unfair practices. Auditing involves analyzing the data, algorithms, and decision-making processes to ensure that they comply with fairness standards. Regular audits promote transparency and ensure accountability in AI development.
โ
During an audit, the data used for training AI algorithms can be thoroughly examined to identify any biases or underrepresentation of certain groups. The algorithms themselves can be scrutinized to ensure that they are not inadvertently discriminating against any particular demographic.
โ
Furthermore, the decision-making processes can be audited to ensure that they are fair, explainable, and in line with ethical guidelines. Auditing can also involve seeking feedback from users or stakeholders to identify any unintended consequences or negative impacts of AI systems.
โ
By conducting regular audits, organizations can demonstrate their commitment to accountability and address any issues or concerns before they escalate. Auditing serves as a crucial mechanism to maintain trust in AI systems and ensure that they are developed and deployed responsibly.
โ
โ
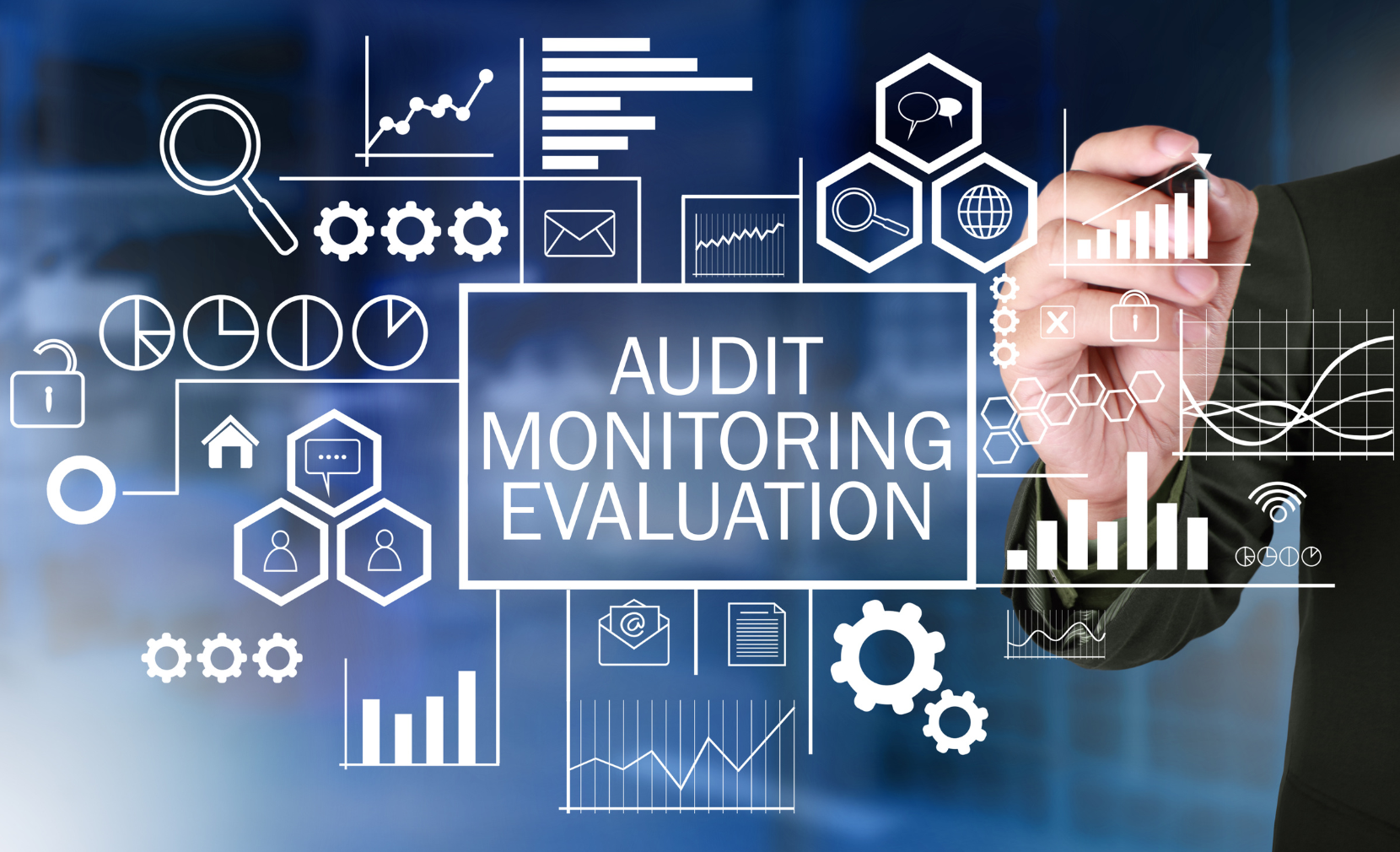
โ
โ
Promoting Transparency in AI Systems
โ
Openness in AI Design and Decision-making
โ
Openness in AI design and decision-making involves making the underlying processes and mechanisms of AI systems accessible to users and stakeholders. This includes sharing information about training data, algorithms used, and decision-making criteria. Openness fosters trust and allows individuals to understand and challenge AI decisions.
โ
Communicating AI Decisions Effectively
โ
Effective communication of AI decisions is important to ensure transparency. AI systems should provide explanations for their decisions in a clear and understandable manner. This includes providing information on the factors considered and the reasoning behind AI decisions.
โ
The Role of Documentation in AI Transparency
โ
Documentation of AI systems plays a crucial role in promoting transparency. Detailed documentation should be maintained, covering aspects such as data sources, data preprocessing techniques, algorithm selection, and decision-making criteria. This documentation serves as a reference for users, regulators, and auditors to understand the processes and decisions made by AI systems.
โ
Conclusion
โ
Building an AI that is fair, accountable, and transparent requires a comprehensive approach. It involves understanding the importance of fairness, accountability, and transparency in AI systems, as well as taking concrete steps to ensure fairness, accountability, and transparency throughout AI development. By integrating these principles into AI design and decision-making, we can create AI systems that benefit society as a whole and earn the trust of users.