In today's world, image recognition has become an indispensable part of our daily lives. From facial recognition on smartphones to automated surveillance systems, we rely on this technology more than we realize. However, image recognition has come a long way from its humble beginnings, evolving into something much more powerful and sophisticated known as image understanding. In this article, we'll explore the birth of image recognition, the transition to image understanding, the differences between the two, and also delve into the future possibilities of visual reasoning.
โ
The Birth of Image Recognition
โ
When image recognition first emerged, it was a relatively simple process. Early techniques primarily focused on identifying specific patterns and features within an image. These early methods lacked the contextual understanding required for more complex tasks.
โ
As technology advanced, researchers and scientists delved deeper into the field of image recognition, seeking to develop more sophisticated techniques. They recognized the need for algorithms that could not only identify patterns but also comprehend the content and context of an image.
โ
Early Techniques in Image Recognition
โ
One of the earliest techniques used in image recognition was template matching. This method involved comparing the image with predefined templates to find a match. While this approach had its limitations, it laid the groundwork for further advancements in the field.
โ
Template matching was a significant step forward in image recognition, as it allowed computers to identify specific objects or patterns within an image. However, it was limited in its ability to handle variations in lighting, scale, and perspective. Researchers soon realized that a more robust and adaptable approach was needed.
โ
The Role of Machine Learning in Image Recognition
โ
The introduction of machine learning revolutionized image recognition. Rather than relying on pre-defined templates, machine learning algorithms enabled computers to learn from large datasets. This breakthrough allowed for more accurate and adaptable recognition systems.
โ
Machine learning algorithms, such as neural networks, were trained on vast amounts of labeled data, allowing them to recognize patterns and features in images with remarkable accuracy. These algorithms could automatically extract relevant features from images, making them more capable of handling variations and complexities.
โ
With the advent of deep learning, a subfield of machine learning, image recognition took another leap forward. Deep learning algorithms, particularly convolutional neural networks (CNNs), became the go-to method for image recognition tasks. CNNs excel at extracting hierarchical features from images, mimicking the human visual system's ability to process information.
โ
The combination of machine learning and deep learning techniques paved the way for groundbreaking applications of image recognition. From facial recognition in security systems to object detection in autonomous vehicles, the capabilities of image recognition continue to expand.
โ
As the field progresses, researchers are exploring new avenues, such as transfer learning and generative adversarial networks (GANs), to further enhance image recognition systems. These advancements hold the promise of even more accurate, efficient, and versatile image recognition in the future.
โ
โ
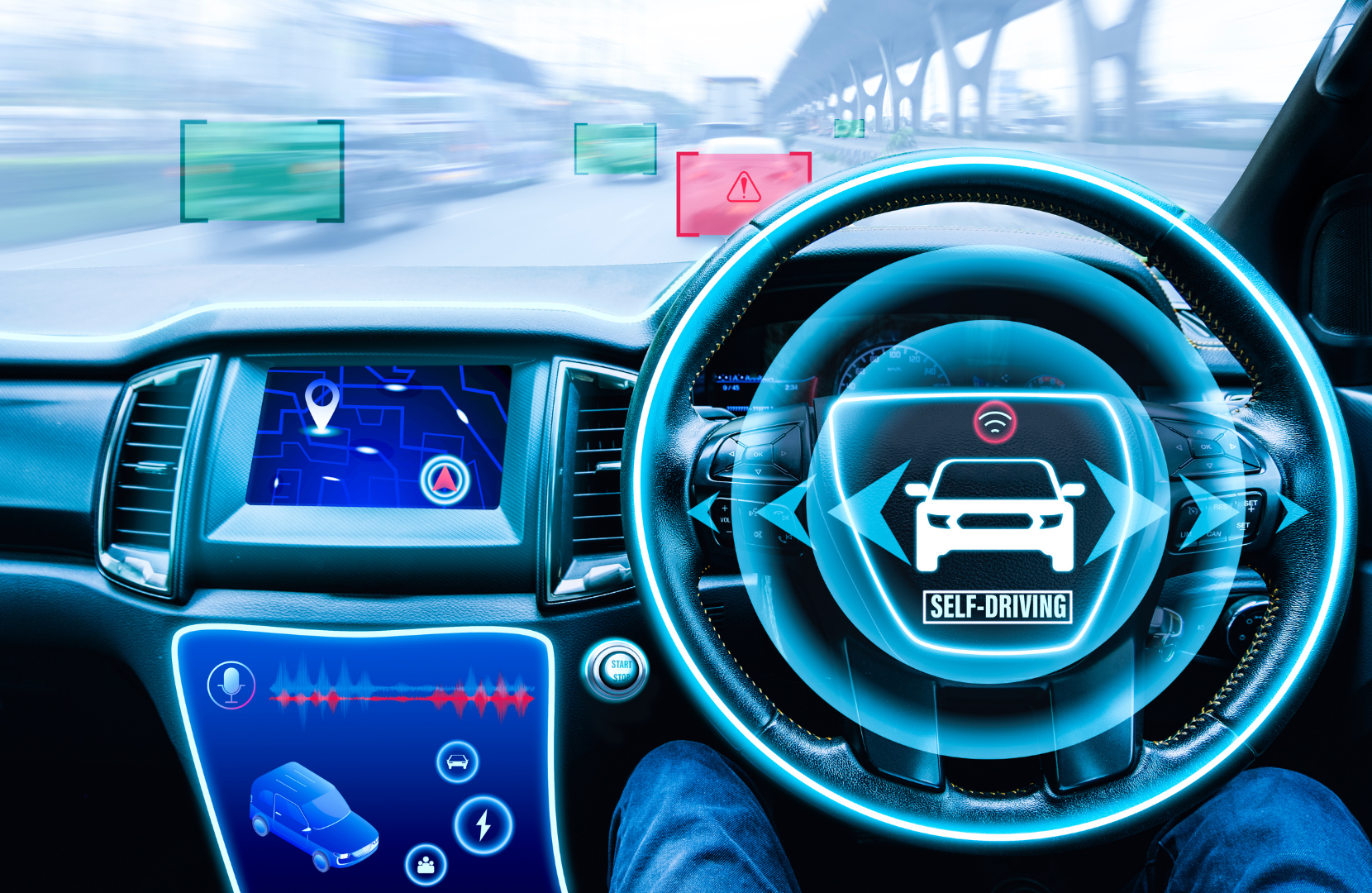
โ
โ
The Evolution to Image Understanding
โ
As technology advanced, image recognition began to transition into image understanding. The concept of visual reasoning emerged, which aimed to mimic human-level understanding of images.
โ
Visual reasoning involves the ability to not only recognize objects within an image but also understand the relationships between them. This higher level of comprehension enabled computers to analyze images in a way that was closer to how humans process visual information.
โ
One of the key challenges in image understanding was the ability to interpret the context and semantics of an image. While early image recognition systems could identify objects, they struggled to understand the meaning behind them. For example, a system might recognize a dog in an image, but it would not be able to understand that the dog is playing with a ball.
โ
To address this challenge, researchers turned to deep learning, a subset of machine learning that focuses on training artificial neural networks with multiple layers. Deep neural networks enabled computers to analyze images at multiple levels of abstraction, allowing for more nuanced interpretations and accurate understanding.
โ
Deep learning models, such as convolutional neural networks (CNNs), revolutionized image understanding by automatically learning hierarchical representations of visual data. These models were trained on large datasets, consisting of millions of labeled images, to learn the complex patterns and relationships between different objects and scenes.
โ
By leveraging the power of deep learning, image understanding systems became capable of not only recognizing objects but also understanding their attributes, such as their size, color, and shape. They could also infer the spatial relationships between objects, such as whether one object is on top of another or if two objects are in close proximity.
โ
Furthermore, deep learning models enabled image understanding systems to perform more advanced tasks, such as object detection, segmentation, and even image captioning. Object detection algorithms could identify and localize multiple objects within an image, while segmentation algorithms could separate different objects from the background. Image captioning systems could generate natural language descriptions of images, providing a human-like understanding of visual content.
โ
With the advancements in deep learning and the concept of visual reasoning, image understanding has made significant progress in recent years. However, there are still many challenges to overcome, such as understanding images in complex and dynamic environments, handling occlusions and ambiguities, and reasoning about abstract concepts. Researchers continue to explore new techniques and approaches to further improve the capabilities of image understanding systems.
โ
โ

โ
โ
The Difference Between Image Recognition and Image Understanding
โ
While image recognition and image understanding may seem similar, they have distinct differences in terms of their processes and capabilities.
โ
Image recognition focuses on identifying and classifying objects within an image. It relies on patterns and features to make predictions. By analyzing the visual characteristics of an image, such as color, shape, and texture, image recognition algorithms can determine what objects are present in the image. This process involves comparing the features extracted from the image to a pre-trained model that contains information about different objects. Once the objects are recognized, they can be labeled or categorized accordingly.
โ
On the other hand, image understanding goes beyond recognition and aims to comprehend the context, relationships, and meaning within an image. It involves a deeper level of analysis and interpretation. Image understanding algorithms not only identify objects but also try to understand their spatial arrangement, their interactions with other objects, and the overall scene composition. This requires a more comprehensive understanding of the visual content and often involves reasoning and inference.
โ
Comparing the Processes: Recognition vs Understanding
โ
Image recognition and image understanding differ in their underlying processes. While image recognition focuses on the identification and classification of objects, image understanding involves a more holistic approach to comprehend the entire image.
โ
When it comes to image recognition, the process typically involves several steps. First, the image is preprocessed to enhance its quality and extract relevant features. These features are then compared to a database of known objects, and the closest matches are identified. Finally, the recognized objects are labeled or categorized based on the information stored in the database.
โ
In contrast, image understanding requires a more complex set of processes. It starts with the initial recognition of objects, similar to image recognition. However, it goes beyond that by analyzing the relationships between objects and their context within the image. This involves understanding the spatial layout, the semantic connections, and the overall meaning of the visual content. Image understanding algorithms often incorporate techniques from computer vision, natural language processing, and machine learning to achieve a deeper level of comprehension.
โ
The Limitations of Image Recognition
โ
Although image recognition has made great strides, it still has its limitations. Recognizing objects in complex environments or understanding abstract concepts remains challenging for current systems. Image recognition algorithms heavily rely on the availability of labeled training data, which limits their ability to recognize objects that are not well-represented in the training set.
โ
Furthermore, image recognition algorithms may struggle with objects that have variations in appearance, such as different angles, lighting conditions, or occlusions. They may also struggle with objects that are similar in shape or color, making it difficult to distinguish between them accurately.
โ
Another limitation of image recognition is its inability to understand abstract concepts or infer higher-level meanings from an image. While it can identify individual objects, it may not be able to grasp the overall context or interpret the intentions behind the visual content.
โ
Despite these limitations, image recognition continues to advance, driven by advancements in deep learning and computer vision research. As researchers develop more sophisticated algorithms and datasets, the capabilities of image recognition are expected to improve, eventually bridging the gap between recognition and understanding.
โ
โ
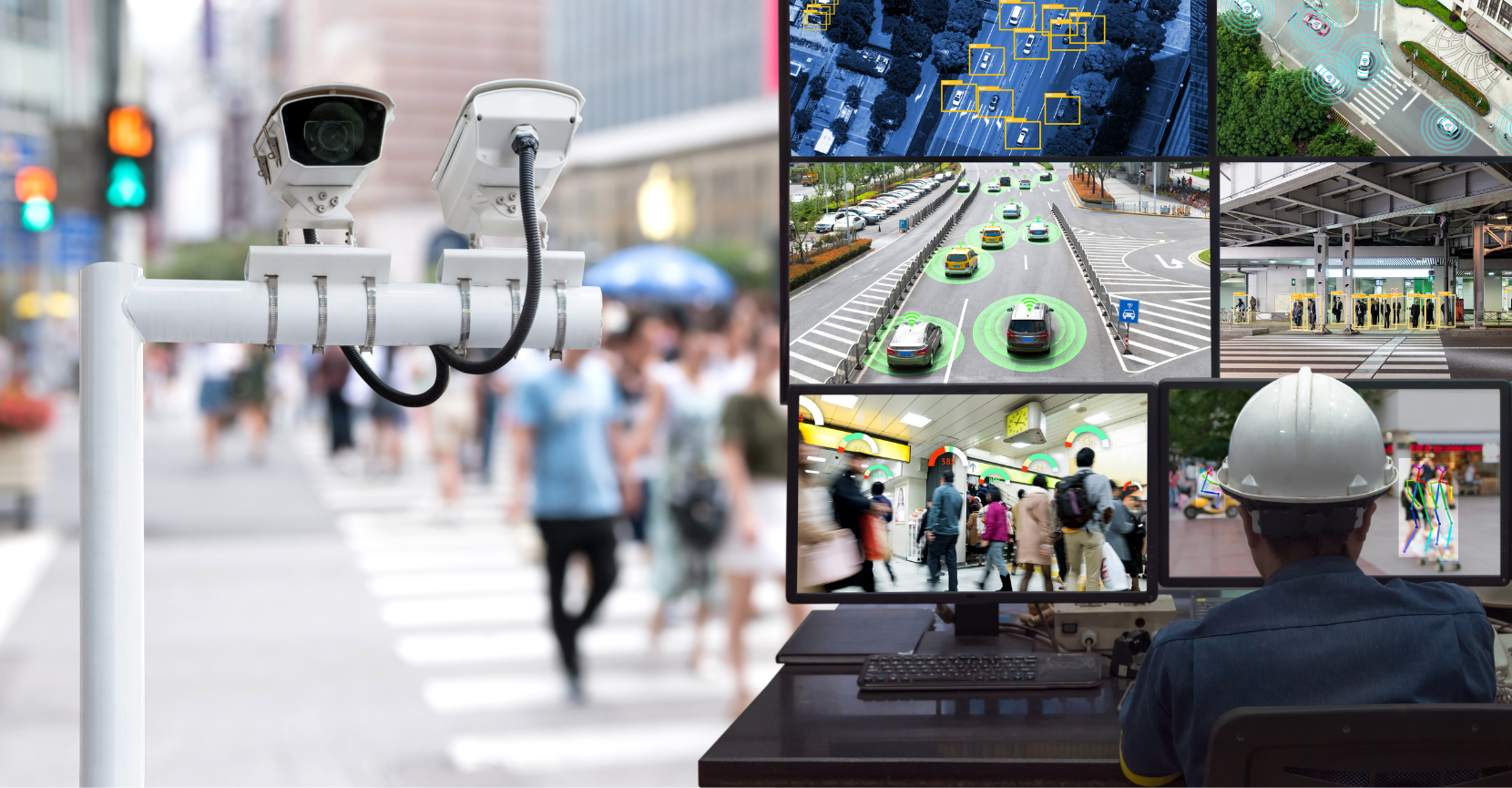
โ
โ
The Future of Visual Reasoning
โ
Looking ahead, the future of visual reasoning is filled with exciting possibilities. Advancements in image understanding have the potential to impact various industries and reshape the way we interact with technology.
โ
Predicted Advancements in Image Understanding
โ
Researchers are continually exploring new techniques for enhancing image understanding. This includes incorporating contextual information, reasoning capabilities, and even integrating other sensory inputs for a more comprehensive understanding of images.
โ
The Potential Impact on Various Industries
โ
Industries such as healthcare, autonomous vehicles, and retail can greatly benefit from the advancements in image understanding. Enhanced visual reasoning capabilities can lead to improved medical diagnoses, safer transportation systems, and more personalized shopping experiences.
โ
Conclusion
โ
Image recognition has evolved into something much more profound: image understanding. With the advent of visual reasoning and the influence of deep learning, computers are slowly but surely gaining the ability to comprehend and interpret visual information. As these technologies continue to advance, we can expect further breakthroughs and exciting applications that will shape our future.