AI Reinforcement Learning (RL) is a subfield of artificial intelligence that has garnered significant attention in recent years. It combines the principles of machine learning and decision-making to create intelligent systems capable of learning from their environment and taking actions based on specific goals. In this article, we will explore the fascinating world of AI RL, its real-life applications, benefits, challenges, and future prospects.
Understanding AI Reinforcement Learning
In order to understand AI reinforcement learning, it is crucial to grasp the concept of reinforcement learning itself. Reinforcement learning is a type of machine learning where an agent interacts with an environment and learns to make decisions by receiving feedback in the form of rewards or penalties. Through this iterative process, the agent continuously adapts its behavior to maximize the rewards it receives.
What is AI Reinforcement Learning?
AI reinforcement learning takes this concept a step further by using artificial intelligence techniques to enable machines to perform complex tasks that require decision-making in real-time environments. By applying RL algorithms, AI systems can learn, adapt, and improve their performance over time.
The Science Behind AI Reinforcement Learning
The science behind AI reinforcement learning is rooted in the field of neuroscience. Inspired by the way animals and humans learn from their experiences, RL algorithms aim to simulate the learning process in machines. By mimicking the brain's reward-based learning approach, AI RL has the potential to achieve remarkable results in a wide range of domains.
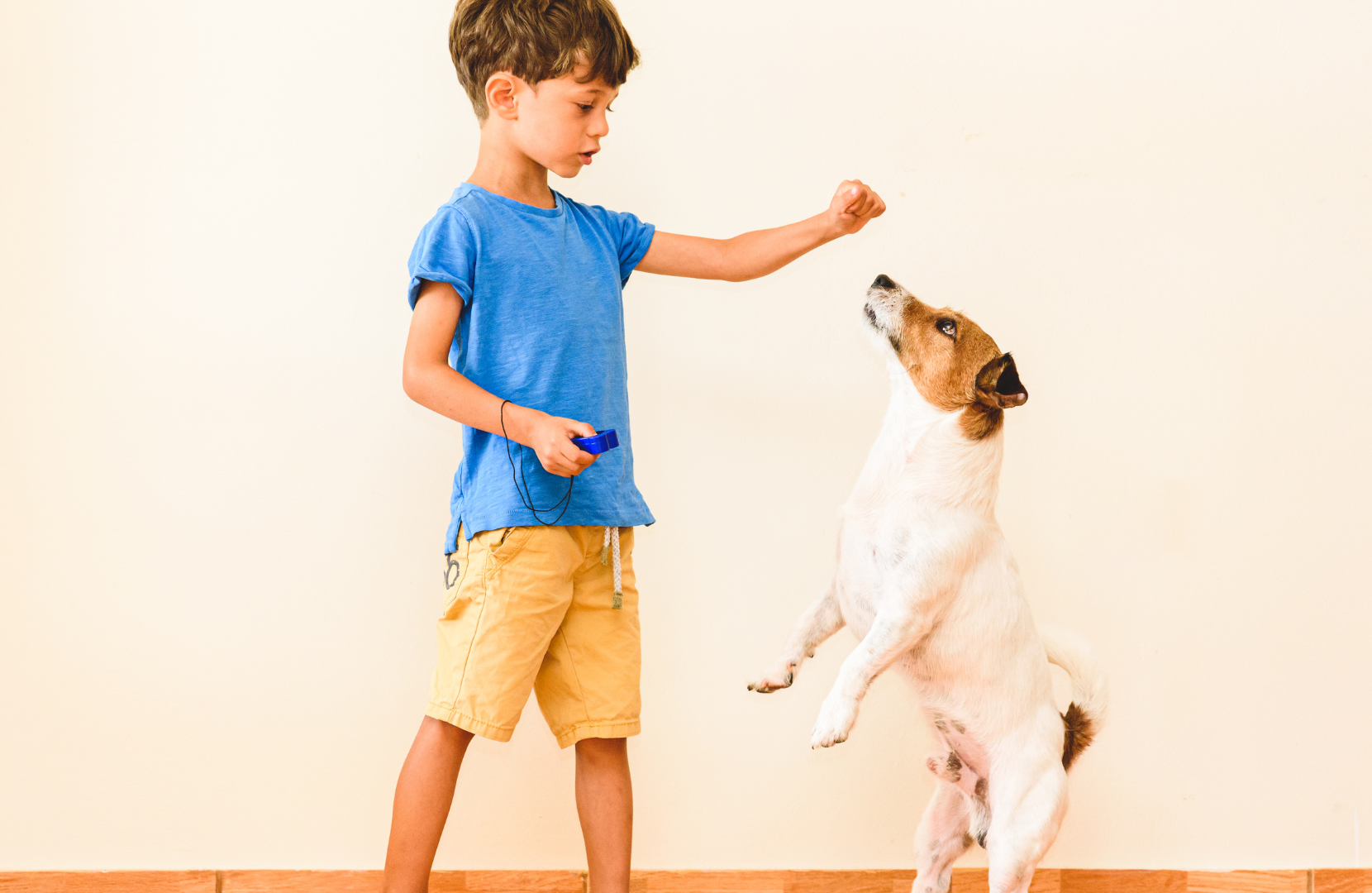
The Evolution of AI Reinforcement Learning
Over the years, the field of AI reinforcement learning has undergone significant advancements. From early experiments with simple board games to state-of-the-art algorithms conquering complex tasks, such as playing Atari games and mastering the game of Go, RL has come a long way. This evolution has paved the way for the application of RL in various real-life scenarios.
Real-Life Applications of AI Reinforcement Learning
The applications of AI reinforcement learning span across multiple industries, bringing forth remarkable advancements and new possibilities.
Healthcare
One industry where AI reinforcement learning has the potential to revolutionize is healthcare. In healthcare, AI reinforcement learning can greatly impact medical diagnosis, treatment planning, and drug discovery. By learning from vast amounts of patient data and expert knowledge, RL systems can assist doctors in making accurate diagnoses and personalized treatment recommendations. This can lead to more effective and efficient healthcare outcomes, ultimately improving patient care and saving lives.
Automotive
Another industry that heavily relies on AI reinforcement learning is autonomous vehicles. The development of autonomous vehicles requires sophisticated algorithms to navigate real-world scenarios. RL algorithms enable vehicles to learn from their driving experiences, making them better equipped to navigate complex traffic situations, avoid accidents, and optimize fuel efficiency. This technology has the potential to revolutionize transportation, making it safer, more efficient, and more accessible to all.

Gaming
Gaming is yet another domain where AI reinforcement learning has made significant strides. By training AI agents to play games and learn from their mistakes, RL algorithms have achieved human-level performance in games like chess, poker, and even complex multiplayer video games. This has not only pushed the boundaries of gaming but also opened up new opportunities for AI in other areas such as virtual reality, simulation, and training environments.
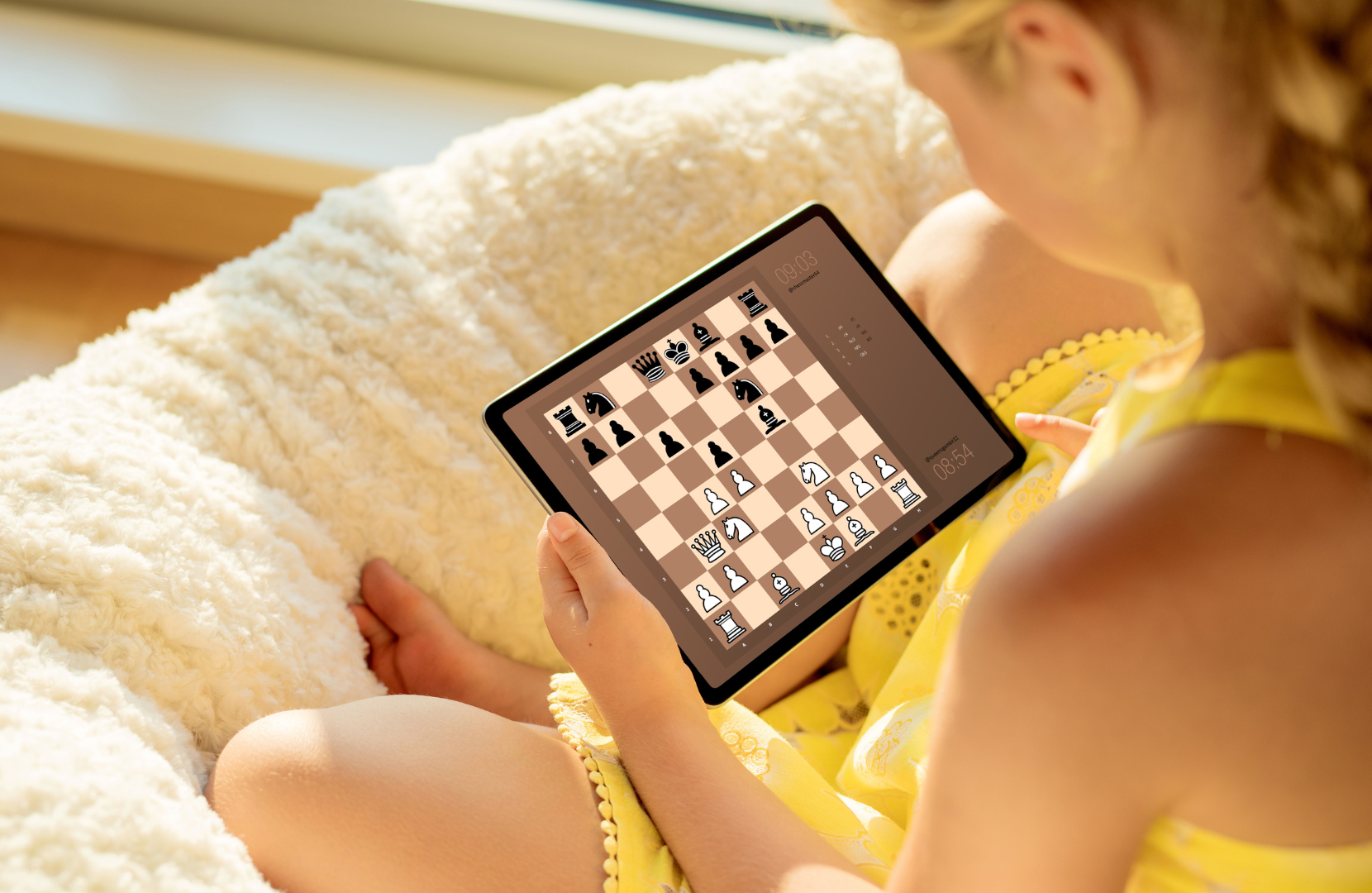
Finance
In the finance industry, AI reinforcement learning has found applications in portfolio management, algorithmic trading, and risk assessment. By learning from historical market data and constantly adapting to changing market conditions, RL-based systems can make smarter investment decisions and mitigate risks. This can lead to improved financial performance, increased efficiency, and reduced human error in financial operations.
These are just a few examples of how AI reinforcement learning is transforming various industries. With continued research and development, we can expect to see even more exciting applications in the future. The potential of AI reinforcement learning is vast, and its impact on society is only just beginning to be fully realized.
The Benefits and Challenges of AI Reinforcement Learning
AI reinforcement learning is a rapidly advancing field that offers great potential for solving complex problems. However, it also presents certain benefits and challenges that need to be carefully considered.
Advantages of Using AI Reinforcement Learning
One of the key advantages of AI reinforcement learning is its ability to learn from experience and optimize performance over time. Unlike traditional rule-based systems, which rely on predefined instructions, RL-based systems can adapt and improve their decision-making abilities through trial and error. This capability makes them well-suited for handling complex and dynamic environments.
For example, in the field of robotics, AI reinforcement learning allows robots to learn how to perform tasks by interacting with their surroundings. By receiving feedback in the form of rewards or penalties, the robots can refine their actions and achieve better performance over time. This flexibility and adaptability make RL-based systems invaluable in industries where the environment is constantly changing.
Another advantage of AI reinforcement learning is its ability to generalize knowledge and apply it to new situations. RL agents can learn abstract concepts and principles that can be transferred to different scenarios. This transfer learning capability enables RL-based systems to quickly adapt to new tasks or environments without the need for explicit programming.
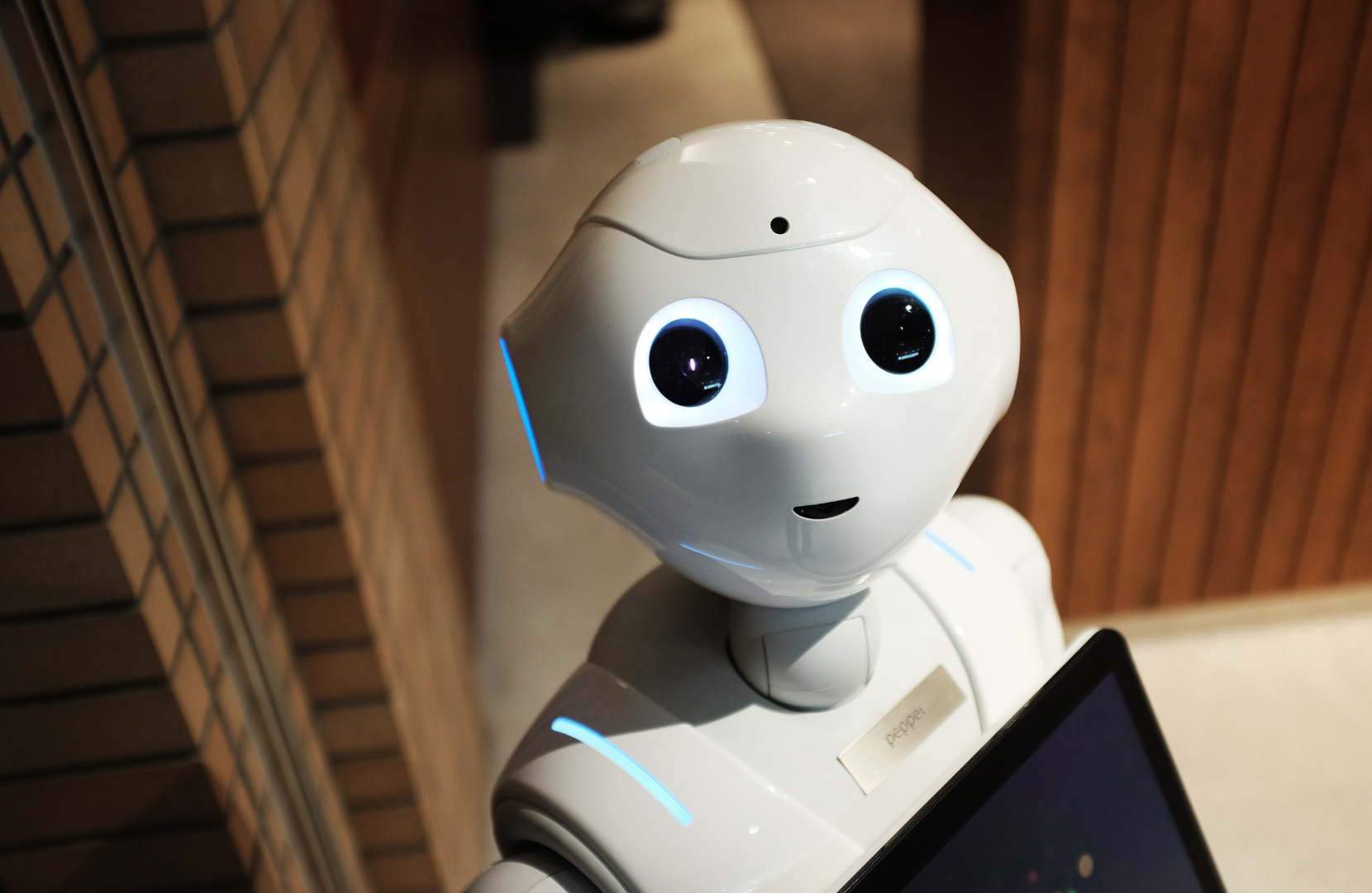
Potential Drawbacks and Challenges
Despite its numerous advantages, AI reinforcement learning also presents certain challenges that need to be addressed for its widespread adoption.
One of the main challenges is the computational intensity and time-consuming nature of training RL models. Reinforcement learning often requires a large amount of data and extensive computational resources to train models effectively. This can be a barrier for organizations with limited resources or those operating in real-time environments where quick decision-making is crucial.
In addition, the lack of interpretability in the decision-making process of RL agents poses concerns, particularly in high-risk domains such as healthcare and finance. Understanding why an RL agent made a particular decision can be difficult, making it challenging to trust and verify its actions. This lack of interpretability raises ethical concerns and may hinder the adoption of RL-based systems in certain industries.
Furthermore, ensuring the ethical use of RL systems and addressing potential biases are critical challenges that need to be addressed as this technology evolves. RL agents learn from the data they are trained on, and if this data contains biases or reflects societal inequalities, the RL systems may perpetuate or amplify these biases. It is essential to develop mechanisms to detect and mitigate bias in RL models to ensure fair and equitable outcomes.
Future Prospects of AI Reinforcement Learning
The future of AI reinforcement learning looks promising, with several emerging trends and prospects on the horizon.
One of the emerging trends in AI reinforcement learning is the combination of RL with other AI techniques such as deep learning. This integration allows for the creation of more powerful and versatile systems. By combining RL with deep learning, agents are able to handle high-dimensional data and learn from raw sensory inputs. This opens up new avenues for applications in computer vision, natural language processing, and robotics. For example, in computer vision, RL can be used to train agents to recognize and understand complex visual patterns, enabling them to accurately identify objects and make intelligent decisions based on visual inputs. In natural language processing, RL can be used to develop conversational AI agents that can understand and respond to human language in a more natural and nuanced way. In robotics, RL can be used to train robots to perform complex tasks and interact with their environment in a more intelligent and adaptive manner.
The future of AI reinforcement learning holds immense potential across various industries. In healthcare, we can expect RL systems to assist in personalized medicine and drug development. With the ability to learn from patient data and medical research, RL agents can help doctors and researchers identify the most effective treatments for individual patients. This can lead to more targeted and personalized healthcare, improving patient outcomes and reducing healthcare costs. In the field of autonomous vehicles, RL will play a crucial role in making them safer and more efficient. By training autonomous vehicles to make intelligent decisions based on real-time data from sensors, RL can help prevent accidents and optimize routes for better fuel efficiency. This will not only save lives but also reduce traffic congestion and emissions. In the finance industry, AI-powered trading systems that use RL algorithms will become more prevalent. These systems can adapt to market conditions with speed and accuracy, making more informed investment decisions and maximizing returns for investors.
Conclusion
AI reinforcement learning is a powerful technology that has the potential to revolutionize several industries. Its real-life applications in healthcare, autonomous vehicles, gaming, and finance are just a glimpse of the possibilities. While AI RL offers numerous benefits, it also presents challenges that need to be addressed, such as ethical considerations and the potential for job displacement. However, with ongoing advancements and emerging trends, the future of AI RL looks promising, opening up new horizons for intelligent systems and shaping the world we live in.