In recent years, artificial intelligence (AI) has revolutionized many aspects of our lives. From virtual assistants to recommendation systems, AI has become an integral part of our daily routine. One particular aspect of AI that has been gaining attention is Explainable AI - the ability to understand and interpret the decision-making process of AI algorithms. In this article, we will dive deep into the concept of Explainable AI and explore how it powers actionable predictions, ultimately automating insight generation.
โ
Understanding the Concept of Explainable AI
โ
If you've ever interacted with an AI system, you might have wondered how it arrives at its conclusions. Explainable AI aims to answer this question by providing insights into the underlying logic and reasoning of AI algorithms. It goes beyond just making accurate predictions; it provides transparency by giving explanations and justifications for its decisions. This is particularly crucial in contexts where the decisions made by AI systems have significant consequences, such as healthcare, finance, or legal domains.
โ
When it comes to AI, explainability is key. Without understanding the reasoning behind AI decisions, users may be hesitant to trust or rely on AI systems. Explainable AI seeks to bridge this gap by providing human-interpretable explanations, making the decision-making process more transparent and understandable.
โ
The Basics of Explainable AI
โ
At the core of Explainable AI is the notion of interpretability. It focuses on developing models and techniques that can be easily understood by humans. Traditional AI algorithms, like deep learning neural networks, often lack interpretability. They operate as "black boxes," making it challenging to understand why they make the predictions they do. Explainable AI, on the other hand, emphasizes the need for algorithms that provide clear explanations of their decision processes.
โ
One approach to achieving interpretability is through the use of rule-based models. These models generate decisions based on a set of predefined rules, making it easier for humans to understand and validate the reasoning behind each decision. Another approach is the use of feature importance techniques, which highlight the most influential factors that contribute to a particular prediction. By understanding which features are driving the decision, users can gain valuable insights into the decision-making process.
โ
โ
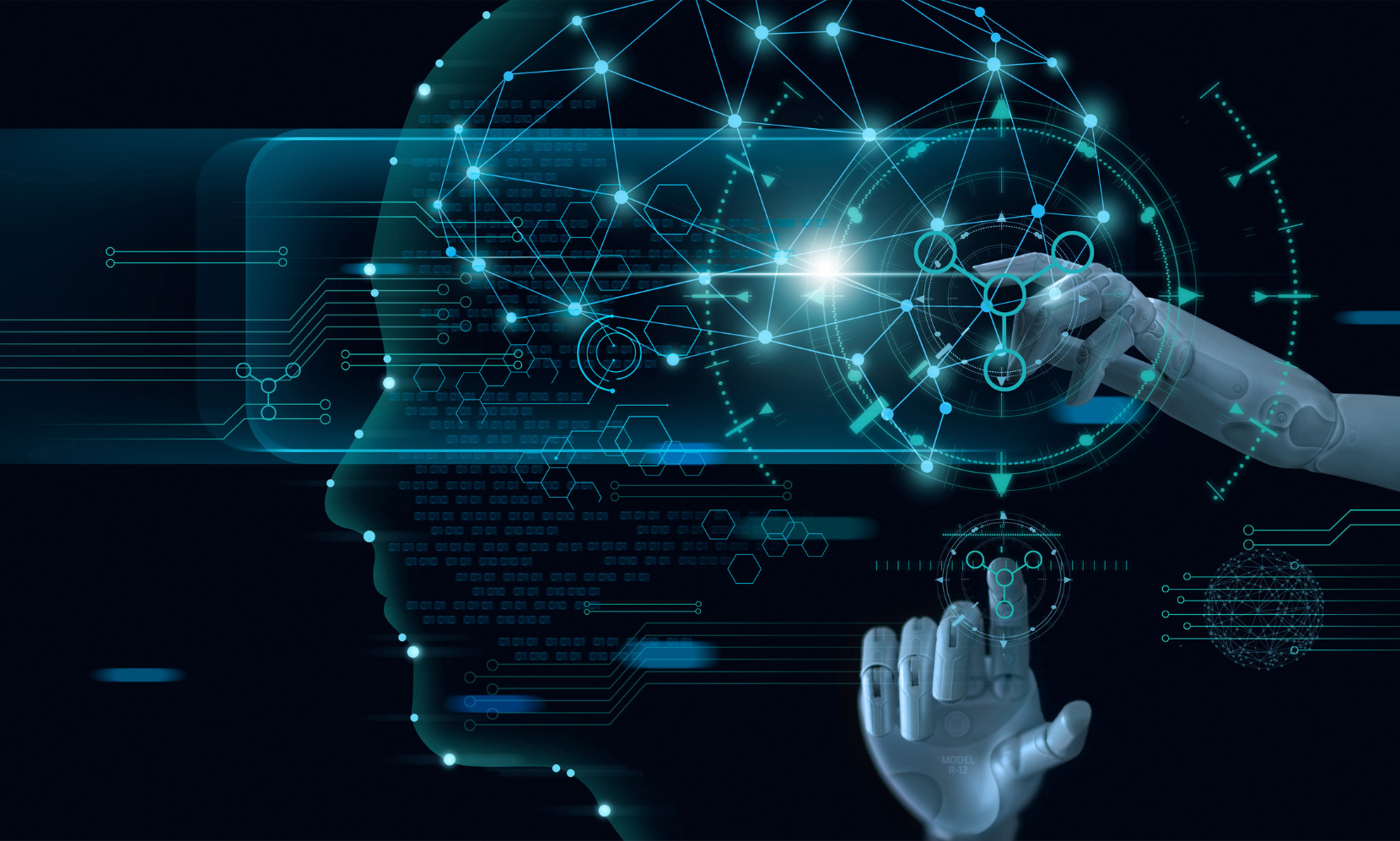
โ
โ
The Role of Explainable AI in Automating Insight Generation
โ
Insight generation is a crucial task in business and various other domains. Traditionally, generating meaningful insights from data required human experts to analyze massive amounts of data manually. However, with the advent of Explainable AI, insight generation can now be automated. By utilizing explainable algorithms, businesses can quickly extract valuable insights from complex data sets, enabling them to make informed decisions more efficiently and effectively.
โ
Explainable AI algorithms can uncover hidden patterns and relationships within data that may not be immediately apparent to human analysts. These algorithms can identify correlations, outliers, and trends, providing valuable insights that can drive business strategies and decision-making. By automating the insight generation process, organizations can save time and resources while gaining a deeper understanding of their data.
โ
Furthermore, explainable AI can help in regulatory compliance and risk assessment. In industries such as finance and healthcare, where regulations and ethical considerations play a significant role, explainable AI provides the necessary transparency to ensure compliance. It allows auditors and regulators to understand and validate the decision-making process, reducing the risk of biased or unfair outcomes.
โ
The Mechanism of Actionable Predictions
โ
Actionable predictions refer to predictions made by AI systems that provide actionable recommendations rather than just static predictions. These predictions go beyond stating what will happen; they provide insights into how to act upon the predicted outcomes. This is a significant step towards making AI systems more valuable in real-world applications.
โ
The Process of Making Predictions with AI
โ
Making predictions with AI involves several stages. First, data is collected and preprocessed, ensuring its quality and suitability for analysis. This data collection process is crucial as it lays the foundation for accurate predictions. The data is carefully curated, ensuring that it represents a diverse range of scenarios and captures the intricacies of the problem at hand.
โ
Once the data is collected, it undergoes preprocessing. This step involves cleaning the data, removing any outliers or errors, and transforming it into a format suitable for analysis. Data preprocessing is essential to ensure that the AI model receives high-quality input, as the accuracy of predictions heavily relies on the quality of the data.
โ
After the data is preprocessed, machine learning algorithms come into play. These algorithms are trained on the preprocessed data to create models capable of making predictions. The training process involves feeding the algorithms with input data and the corresponding correct output. The algorithms learn from this data, identifying patterns and relationships that allow them to make accurate predictions.
โ
Once the models are trained, they can be used to make predictions. Input data is fed into the models, and they generate output predictions based on the patterns and relationships they have learned during the training process. These predictions can provide valuable insights into future outcomes, helping businesses and individuals make informed decisions.
โ
โ

โ
โ
The Importance of Actionable Predictions in Business
โ
Actionable predictions hold immense value in business settings. Imagine if you could accurately predict customer behavior or market trends and receive actionable recommendations on how to adapt your strategies accordingly. This would undoubtedly give businesses a significant advantage by allowing them to proactively respond to changing dynamics, optimize operations, and increase overall profitability.
โ
For example, in the retail industry, actionable predictions can help businesses optimize their inventory management. By accurately predicting customer demand, businesses can ensure that they have the right products in stock at the right time, avoiding stockouts or overstocking. This not only improves customer satisfaction but also reduces costs associated with inventory management.
โ
In the healthcare sector, actionable predictions can revolutionize patient care. By analyzing patient data and predicting disease progression, healthcare providers can intervene early and provide personalized treatment plans. This can lead to improved patient outcomes and reduced healthcare costs.
โ
Actionable predictions also play a crucial role in financial services. By accurately predicting market trends and customer behavior, financial institutions can make informed investment decisions and offer personalized financial advice to their clients. This can help individuals and businesses make better financial choices and achieve their financial goals.
โ
The Intersection of Explainable AI and Insight Generation
โ
The combination of Explainable AI and insight generation brings even more value to businesses. Explainable AI algorithms can not only generate insights but also provide explanations and justifications for those insights. This enables stakeholders to have a deeper understanding of the underlying factors driving the insights, improving trust and confidence in the decision-making process.
โ
How Explainable AI Facilitates Insight Generation
โ
Explainable AI can facilitate insight generation in several ways. By providing clear explanations of how insights are derived, stakeholders can better comprehend the reasoning behind the generated insights. This understanding fosters trust and acceptance, especially in critical decision-making processes. Additionally, Explainable AI can identify and highlight the salient features and factors that contribute to the insights, helping stakeholders better grasp the significance and relevance of the findings.
โ
The Benefits of Automating Insight Generation with AI
โ
Automating insight generation with AI offers numerous benefits. Firstly, algorithms can process vast amounts of data quickly and accurately, reducing the time and effort required for traditional manual analysis. Secondly, by leveraging Explainable AI techniques, businesses can gain a deeper understanding of the insights generated. This empowers them to make informed decisions and take actions based on a thorough understanding of the underlying factors influencing the insights.
โ
โ

โ
โ
Overcoming Challenges in Implementing Explainable AI
โ
While the promise of Explainable AI is significant, there are challenges to overcome when implementing it in practice.
โ
Common Obstacles in AI Implementation
โ
One of the challenges lies in developing algorithms that strike a balance between accuracy and interpretability. Highly complex AI models often sacrifice interpretability in favor of achieving high accuracy. Finding the right trade-off is crucial for Explainable AI to be practical and useful in real-world scenarios.
โ
Strategies for Successful AI Integration
โ
Successful AI integration requires a holistic approach. Organizations must invest in building a culture that embraces AI and values explainability. Additionally, collaboration between AI experts and domain experts is essential to ensure the models developed align with the needs and requirements specific to the domain. Furthermore, focusing on proactive ethics and ensuring responsible AI practices can help overcome challenges and foster trust among stakeholders.
โ
The Future of Explainable AI in Business
โ
As AI continues to advance, the role of Explainable AI in business will become even more critical.
โ
Predicted Trends in AI and Insight Generation
โ
In the future, we can expect AI algorithms to become more transparent and interpretable. Explainable AI techniques will likely become more mainstream, enabling businesses to gain deeper insights into the decision-making process of AI models while maintaining high accuracy levels. This will drive broader adoption of AI across various industries and lead to more informed decision-making and improved outcomes.
โ
Preparing Your Business for the AI Revolution
โ
To prepare for the AI revolution, businesses need to invest in AI talent, infrastructure, and data capabilities. By embracing Explainable AI and leveraging its benefits in automating insight generation, organizations can position themselves as leaders in their respective industries. The key is to build a strong foundation of AI capabilities and foster a culture that values explainability, ethics, and continuous learning.
โ
Conclusion
โ
Automating Insight Generation: How Explainable AI Powers Actionable Predictions is poised to transform the way businesses generate insights and make decisions. By embracing Explainable AI, organizations can unleash the full potential of AI by not only making accurate predictions but also providing transparent explanations. Through this combination, businesses can empower themselves to act upon these predictions in a proactive and informed manner, driving growth and success in an increasingly data-driven world.