Traditional sensors have played a crucial role in various technologies over the years. They have allowed us to measure and monitor physical quantities such as temperature, pressure, and light intensity. However, these conventional sensing methods have their limitations. Thankfully, with the advent of statistical machine learning, we are now able to go beyond the capabilities of traditional sensors and unlock a whole new world of advanced sensing technologies.
โ
Understanding Traditional Sensors and Their Limitations
โ
Sensors are devices that detect and respond to a physical stimulus and convert it into an electrical signal. They have been instrumental in enabling the functionality of many technologies we rely on today. For example, in the automotive industry, sensors are used for engine management, airbag deployment, and antilock braking systems.
โ
However, traditional sensors have certain limitations that hinder their ability to deliver accurate and reliable measurements in all situations. One significant drawback is their inability to adapt to changing environmental conditions. Traditional sensors are often calibrated for a specific range of values and may struggle to provide accurate readings outside of that range. Additionally, they may be vulnerable to noise and interference, leading to inaccurate measurements.
โ
The Role of Sensors in Technology
โ
Sensors are the cornerstone of many technological advancements we see today. They are used in a wide range of applications, including healthcare, transportation, manufacturing, and environmental monitoring. In healthcare, sensors are used in medical devices to monitor vital signs, such as heart rate and blood pressure, allowing for better patient care and timely interventions. In the transportation industry, sensors are used in autonomous vehicles to detect and respond to the surrounding environment, ensuring safe navigation and collision avoidance.
โ
Moreover, sensors play a crucial role in manufacturing processes. They are used to monitor and control various parameters, such as temperature, pressure, and humidity, ensuring optimal conditions for production. This allows for improved quality control and increased efficiency in manufacturing operations. Additionally, sensors are employed in environmental monitoring systems to measure air and water quality, detect pollutants, and monitor climate conditions. These measurements help in assessing the impact of human activities on the environment and aid in the development of sustainable practices.
โ
โ

โ
โ
The Drawbacks of Conventional Sensing Methods
โ
While traditional sensors have undoubtedly revolutionized various industries, they have their limitations. One major drawback is their fixed functionality. Traditional sensors are designed to perform a specific task, and they are not easily adaptable to changing conditions or new requirements. This lack of flexibility can be a significant hindrance in today's fast-paced and continuously evolving world. Additionally, traditional sensing methods often require large amounts of data to be processed and analyzed manually, leading to potential delays and inefficiencies.
โ
Furthermore, traditional sensors may be limited in their ability to provide real-time data. In some applications, such as monitoring critical infrastructure or detecting potential hazards, real-time information is essential for timely decision-making. However, traditional sensors may have latency issues, where there is a delay between the occurrence of an event and the sensor's response. This delay can be detrimental in situations where immediate action is required to prevent accidents or mitigate risks.
โ
Introduction to Statistical Machine Learning
โ
Statistical machine learning is a powerful tool that combines statistical methods with computational algorithms to enable machines to learn and make predictions or decisions without being explicitly programmed. It is a branch of artificial intelligence that focuses on the development of algorithms and models that can learn from and make predictions or decisions based on data.
โ
The Basics of Machine Learning
โ
At its core, machine learning is about building statistical models that can automatically learn patterns in data and make accurate predictions or decisions based on those patterns. To achieve this, machine learning algorithms undergo a training process in which they analyze a large dataset, identify patterns and relationships within the data, and use that knowledge to make predictions or decisions when presented with new, unseen data. This ability to learn from data and generalize to new situations is what sets machine learning apart from traditional programming.
โ
Let's take a closer look at the training process in machine learning. When training a machine learning model, a dataset is divided into two parts: a training set and a testing set. The training set is used to teach the model, allowing it to learn the patterns and relationships in the data. The testing set, on the other hand, is used to evaluate the performance of the trained model by measuring its ability to make accurate predictions or decisions on unseen data. This separation of data into training and testing sets helps to assess the model's ability to generalize and make accurate predictions on new, unseen data.
โ
One of the key concepts in machine learning is the notion of "features". Features are the variables or attributes of the data that are used to make predictions or decisions. For example, in a model that predicts house prices based on features such as square footage, number of bedrooms, and location, these features would be used to learn the relationship between the input variables and the target variable (house price). By analyzing the patterns and relationships between the features and the target variable, the model can make accurate predictions on new, unseen data.
โ
โ
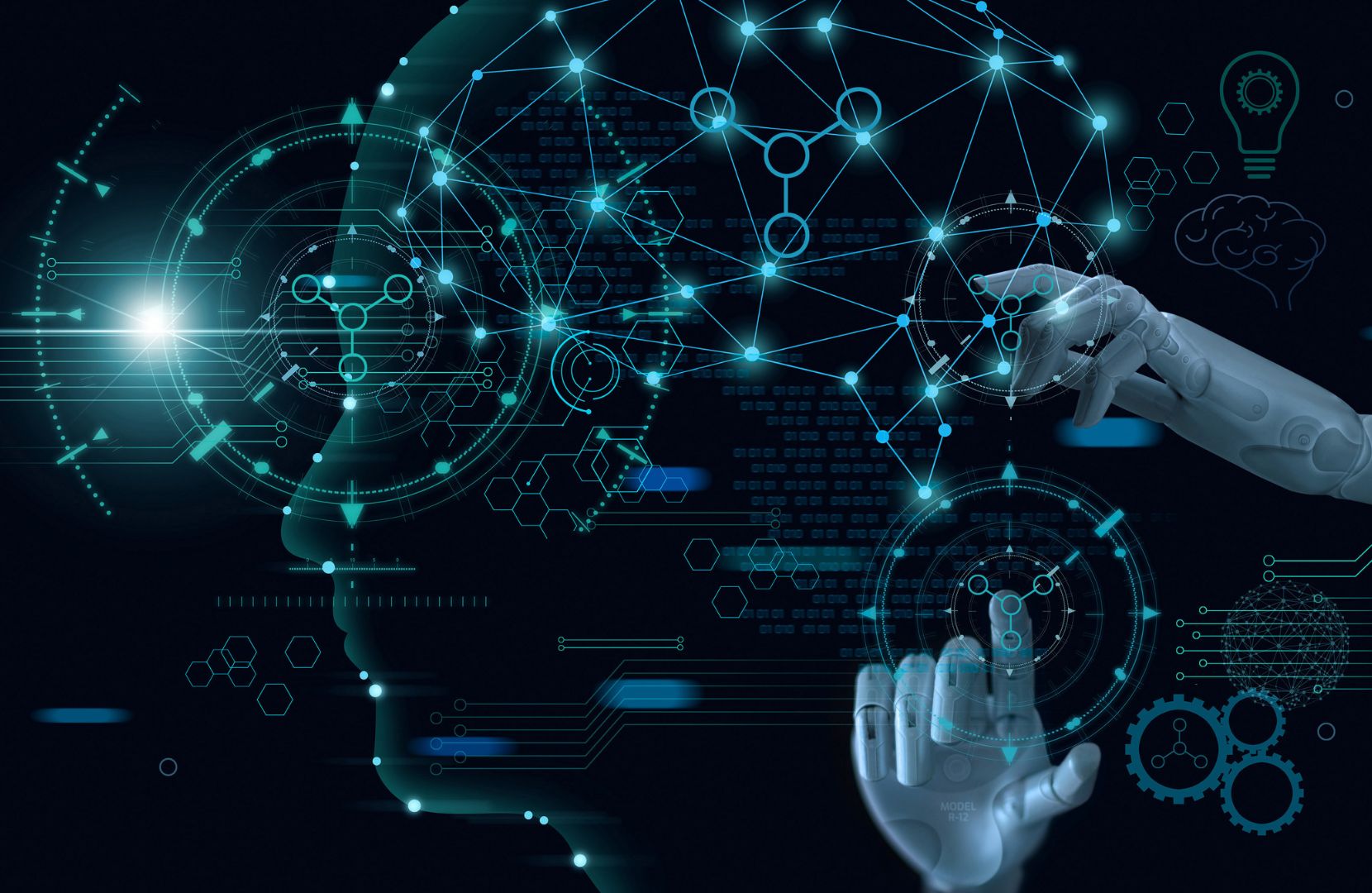
โ
โ
The Importance of Statistics in Machine Learning
โ
Statistics plays a vital role in machine learning, providing the mathematical framework for understanding uncertainty, making inferences, and evaluating model performance. Statistical techniques, such as regression analysis, hypothesis testing, and probability theory, are used to model relationships between variables, assess the significance of those relationships, and quantify the uncertainty associated with predictions or decisions.
โ
Regression analysis, for example, is a statistical technique used to model the relationship between a dependent variable and one or more independent variables. In machine learning, regression models are often used to predict a continuous target variable based on a set of input features. By fitting a regression model to the training data, the machine learning algorithm can learn the relationship between the input features and the target variable, allowing it to make accurate predictions on new, unseen data.
โ
Hypothesis testing is another important statistical technique used in machine learning. It allows us to assess the significance of relationships between variables and make inferences about the population based on sample data. In machine learning, hypothesis testing can be used to determine whether the relationship between a feature and the target variable is statistically significant, helping to identify the most important features for making accurate predictions or decisions.
โ
Probability theory is also fundamental to machine learning. It provides a mathematical framework for quantifying uncertainty and making predictions based on probabilities. Machine learning algorithms often use probability distributions to model the uncertainty associated with predictions or decisions. By estimating the probabilities of different outcomes, the algorithm can make informed decisions and assess the confidence of its predictions.
โ
Bridging the Gap: Machine Learning in Sensing Technologies
โ
With our understanding of traditional sensors and the basics of machine learning, we can now explore how statistical machine learning bridges the gap between the two, revolutionizing the world of sensing technologies.
โ
The Evolution of Sensing Technologies
โ
Sensing technologies have come a long way since the invention of traditional sensors. We have witnessed advancements in sensor design, miniaturization, and integration, making sensors more compact, energy-efficient, and capable of measuring a broader range of physical quantities. These advancements have laid the foundation for the integration of machine learning algorithms into sensing technologies, enabling them to adapt and learn from their environment.
โ
โ
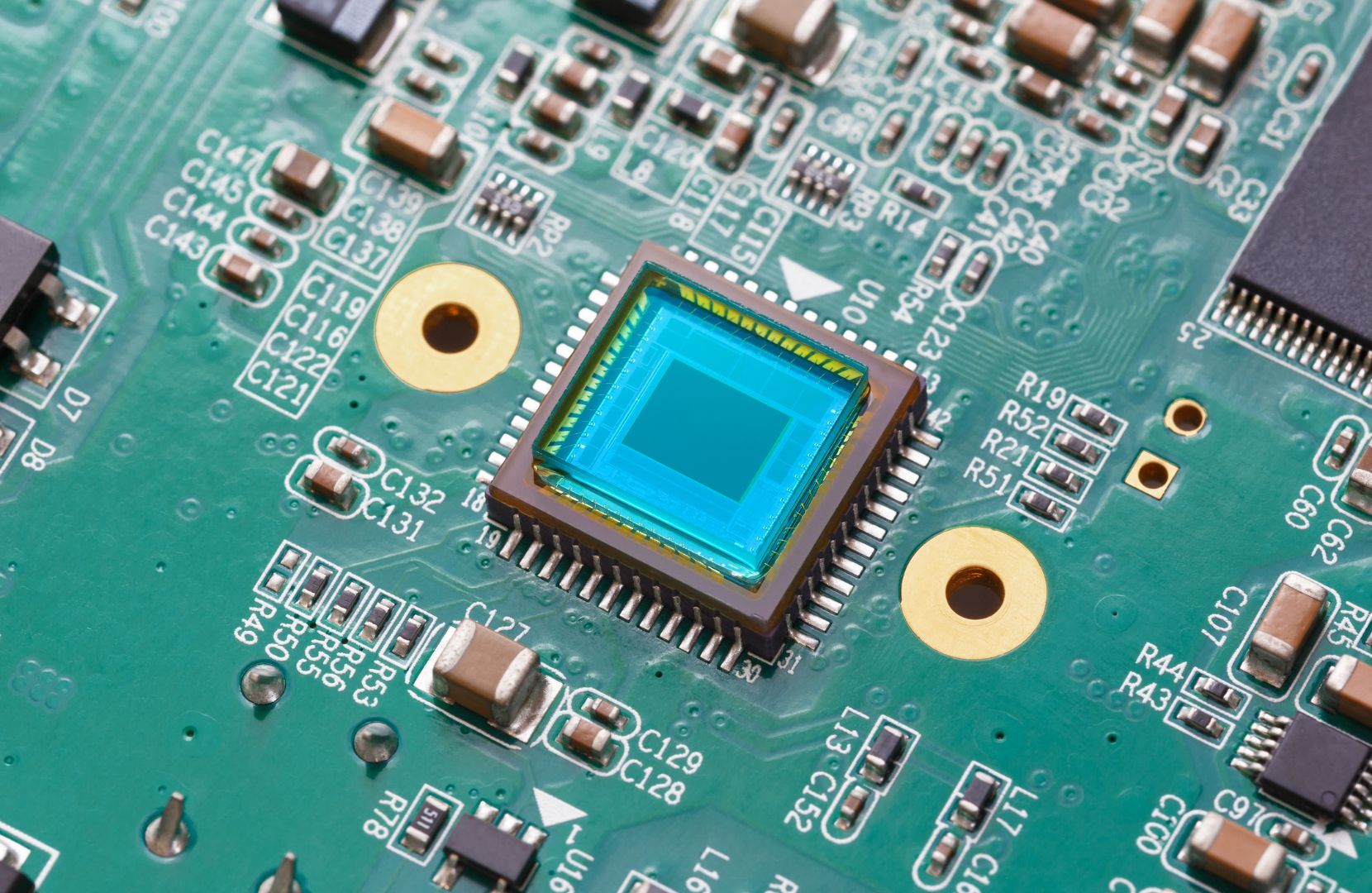
โ
โ
How Machine Learning Enhances Sensor Capabilities
โ
Machine learning algorithms can enhance sensor capabilities in several ways. Firstly, they can improve the accuracy and reliability of measurements by learning from historical data and adapting to changing conditions. By analyzing patterns in the data, machine learning algorithms can compensate for sensor drift, calibration errors, and other sources of measurement inaccuracies.
โ
Additionally, machine learning algorithms can enable sensors to perform advanced tasks such as anomaly detection, predictive maintenance, and event recognition. By learning from data collected by the sensors, these algorithms can identify patterns and anomalies that may not be apparent to traditional sensors alone. This opens up new possibilities for proactive maintenance, early detection of malfunctions, and optimization of operational processes.
โ
The Impact of Advanced Sensing Technologies
โ
The integration of statistical machine learning with advanced sensing technologies has the potential to revolutionize various industries and improve our day-to-day lives in numerous ways.
โ
Benefits of Machine Learning-Driven Sensors
โ
Machine learning-driven sensors offer numerous benefits over traditional sensors. Firstly, they provide more accurate and reliable measurements by leveraging historical data and adapting to changes in the environment. This improved accuracy can lead to better decision-making, increased efficiency, and reduced costs.
โ
Moreover, machine learning-driven sensors can unlock new insights and opportunities by analyzing vast amounts of data in real-time. They can identify patterns, correlations, and anomalies that may not be evident to human operators or traditional sensors. This can enable organizations to optimize processes, improve product quality, and identify potential risks or opportunities.
โ
Potential Challenges and Solutions
โ
While the integration of machine learning in sensing technologies brings forth many advantages, it also presents some challenges. One such challenge is the need for large amounts of high-quality training data to train machine learning algorithms effectively. Gathering and annotating such datasets can be time-consuming and resource-intensive. Additionally, the interpretability of machine learning models can be a concern. As the complexity of the models increases, it becomes more challenging to understand and explain the reasoning behind their predictions or decisions.
โ
However, ongoing research and advancements in data collection, pre-processing techniques, and model interpretability are addressing these challenges and paving the way for wider adoption and deployment of machine learning-driven sensing technologies.
โ
Future Trends in Machine Learning and Sensing Technologies
โ
As we look ahead, the future of machine learning and sensing technologies appears promising. Here are a few predictions for what we can expect in the coming years:
โ
Predictions for the Future of Sensing Technologies
โ
Advancements in sensing technologies will continue to drive innovation across industries. We can expect sensors to become even smaller, more energy-efficient, and capable of measuring a broader range of physical quantities. Moreover, the integration of multiple sensors using techniques such as sensor fusion will enable us to gather more comprehensive and accurate information about our environment.
โ
The Role of Machine Learning in Future Developments
โ
Machine learning will play a significant role in shaping the future of sensing technologies. As machine learning algorithms become more sophisticated and efficient, they will unlock new capabilities and insights from the vast amounts of data collected by sensors. Additionally, the deployment of edge computing, where data processing happens locally on the sensor device itself, will further enhance the real-time capabilities of machine learning-driven sensing technologies.
โ
Conclusion
โ
The integration of statistical machine learning with advanced sensing technologies goes beyond traditional sensors' limitations and enables us to unlock new possibilities. By combining statistical methods and computational algorithms, machine learning-driven sensors can provide more accurate and reliable measurements while offering insights and opportunities that were previously untapped. As we look to the future, the continued advancements in machine learning and sensing technologies hold the promise of improving various industries and enhancing our daily lives.