Even if you don’t realize it, artificial intelligence (AI) is used by millions of people every day. From travel and real estate to manufacturing and banking, AI has started to replace cumbersome tasks, complex analyses, and everything in between. If it were to become fully integrated into the healthcare system, innovative technologies like AI and cryonics could revolutionize the industry. But that’s still a long way away. AI has only just begun to infiltrate the healthcare industry and there are still several roadblocks to more intensive applications. Here, we’ll dive deeper into some of the biggest challenges facing AI in healthcare today.Â

‍
Current Applications of AI in Healthcare
‍
AI has become deeply integrated across many major industries. In addition to its use in logistics, entertainment, travel, and e-Commerce, AI is slowly being introduced to medical facilities. Today, AI is used to streamline routine tasks, such as patient billing, categorization, and revenue accounting. AI also assists with facility management.
‍
In more hands-on medical applications, AI can be used to segment X-ray images and make treatment suggestions based on diagnostic testing or data. This improves efficiency, but it doesn’t mean we no longer need doctors or nurses. The current applications of healthcare-related AI primarily involve tasks that make the lives of medical professionals easier and more efficient. They don’t replace the individual.Â
‍
Furthermore, AI tools shouldn’t be confused with robotic assistance. Medical robots are often used in surgery to help reduce incision size, mitigate risk, and decrease scarring, but they’re overseen by a surgeon or doctor. Robotics can also improve prosthetic devices for amputees and help with cleaning and disinfecting. Although all medical robots have integrated artificial intelligence technology, not all AI technology is in the form of a robot.Â
‍

‍
7 Biggest Challenges of AI in Healthcare
‍
While global algorithm-based healthcare solutions are expected to grow from $6.7 billion in 2020 to $120.8 billion by 2028 [10], there are still several challenges that need to be addressed. Not only does AI in healthcare need to uphold ethical standards and protect sensitive patient data, but it also needs to definitively improve patient outcomes in order to be adopted. To further understand the uphill battle that persists, consider some of the following challenges of adopting AI tools in the healthcare industry.Â
‍
Lack of Standardization
‍
As of right now, there’s no standard method for testing, tracking, analyzing, and inputting datasets gathered through AI tools in the healthcare industry. Everyone is trying to create their own AI software to use within their region or facility. This makes it difficult to compare results and improve deep learning processes.Â
‍
What’s more, many healthcare-related AI tools are also either developed by AI researchers who don’t have medical expertise or medical researchers who don’t have AI expertise [6].Â
‍
For future success regarding machine learning in the healthcare industry, standardization of data needs to occur. Researchers need to take a collaborative approach to AI design and work together to improve existing models, rather than trying to develop unique models for personal application. As Technology Review puts it, “the collective effort of researchers around the world produced hundreds of mediocre tools, rather than a handful of properly trained and tested ones” [6].Â
‍
To combat the current lack of standardization, the nonprofit MITRE Corporation proposed a Standard Health Record (SHR). This would outline a specific, high-quality, and computable way of collecting patient information. Unfortunately, due to the high costs of adoption, the incentive to implement the SHR and work together is low.
‍
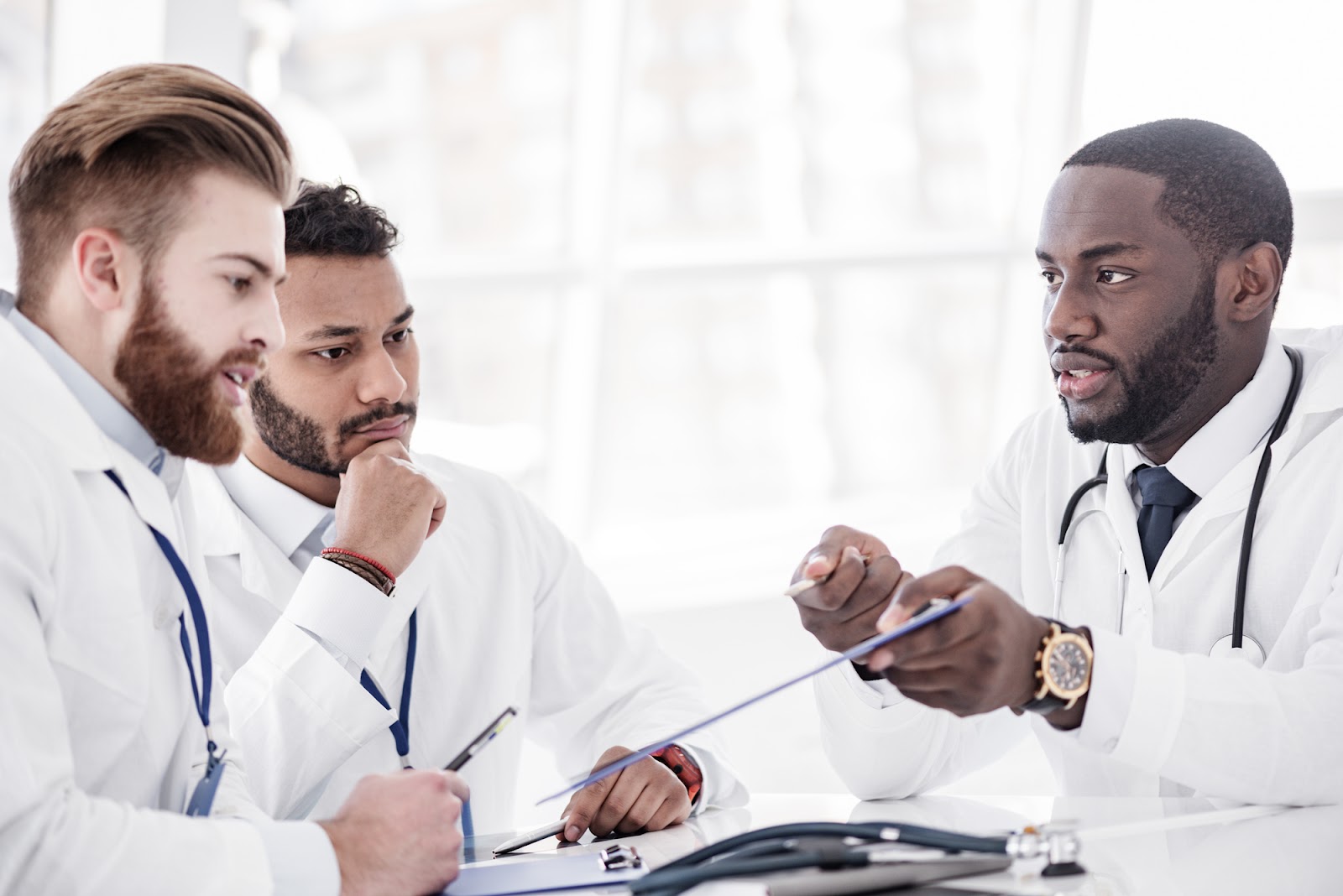
‍
Interoperability Issues
‍
When you search for something online, the results are automated. The process is 100% identical for anyone who makes the same search. Google uses AI for these queries and different keywords will generate different results. This type of interoperability doesn’t currently exist across healthcare facilities.Â
‍
As mentioned, doctors don’t have a standardized method of recording. There’s no systematic way of inputting data into electronic systems or databases. Even if two doctors used the same metrics to measure patient data, details about individual stress levels, sleep, and diet aren’t routinely collected. Yet these factors can impact underlying conditions or diseases.Â
‍
As of right now, this creates incompatible performance metrics for AI applications. The information that’s gathered is neither complete nor uniform across different databases, which can significantly skew the machine learning responses and predictions. When data can’t be interpreted, it can’t be used safely.Â
‍
As of 2019, close to 75% of healthcare facilities indicated that they were “beyond a foundational level of interoperability — that being the ability to exchange data between systems of record but not necessarily being able to interpret the information” [11].Â
‍
For the most effective use of healthcare-based AI, deep learning models and results need to be easily integrated into the workflow of all medical professionals. Medical data needs to be compatible across different platforms to improve interoperability and access.Â
‍

‍
Poor Quality Data for Validating AI
‍
The digitalization of patient files has created a large dataset, but targeted segments are still incredibly small. Clinical trials of AI use within the healthcare sector are also limited, with subjects ranging from 50 to 1,000 patients [1]. Even though data exists for millions of people, after you filter results based on symptoms, metrics, or demographics, you may find the results only encompass a few hundred thousand or less [1]. And that’s the best-case scenario. What usually happens is that data isn’t transferred between facilities, due to lacking interoperability. This further reduces the standardization of research and patient information.
‍
Therefore, one of the biggest challenges in developing and testing potential models for AI in healthcare is the lack of quality data used to develop machine learning tools. When AI is constructed using data from unknown sources or underrepresented individuals, it can skew the deep learning process and therefore, alter results [7].Â
‍
One interesting example of this is when the AI developed for COVID-19 used a data set that, according to an article in Technology Review, “contained chest scans of children who did not have covid as their examples of what non-covid cases looked like” [6]. The result was AI that learned how to identify kids, not covid itself. Â
‍
This is a common problem in AI within the healthcare industry. When machine learning tools use poor-quality data, they don’t obtain an accurate representation of real-world data.Â
‍
They also make mistakes regarding correlation vs. causation. Better data would make a huge impact on the success of future tools. It would create medical AI that isn’t skewed or biased and allow for better predictions based on individual circumstances. Â
‍
One of the best ways to improve data quality is to create a standardized method of collection and analysis. “Connected data lakes,” data without schematic restrictions, could help achieve this [8]. Otherwise, data that are not suitably formatted or documented will continue to hinder the process of healthcare-related AI and its potential applications.Â
‍
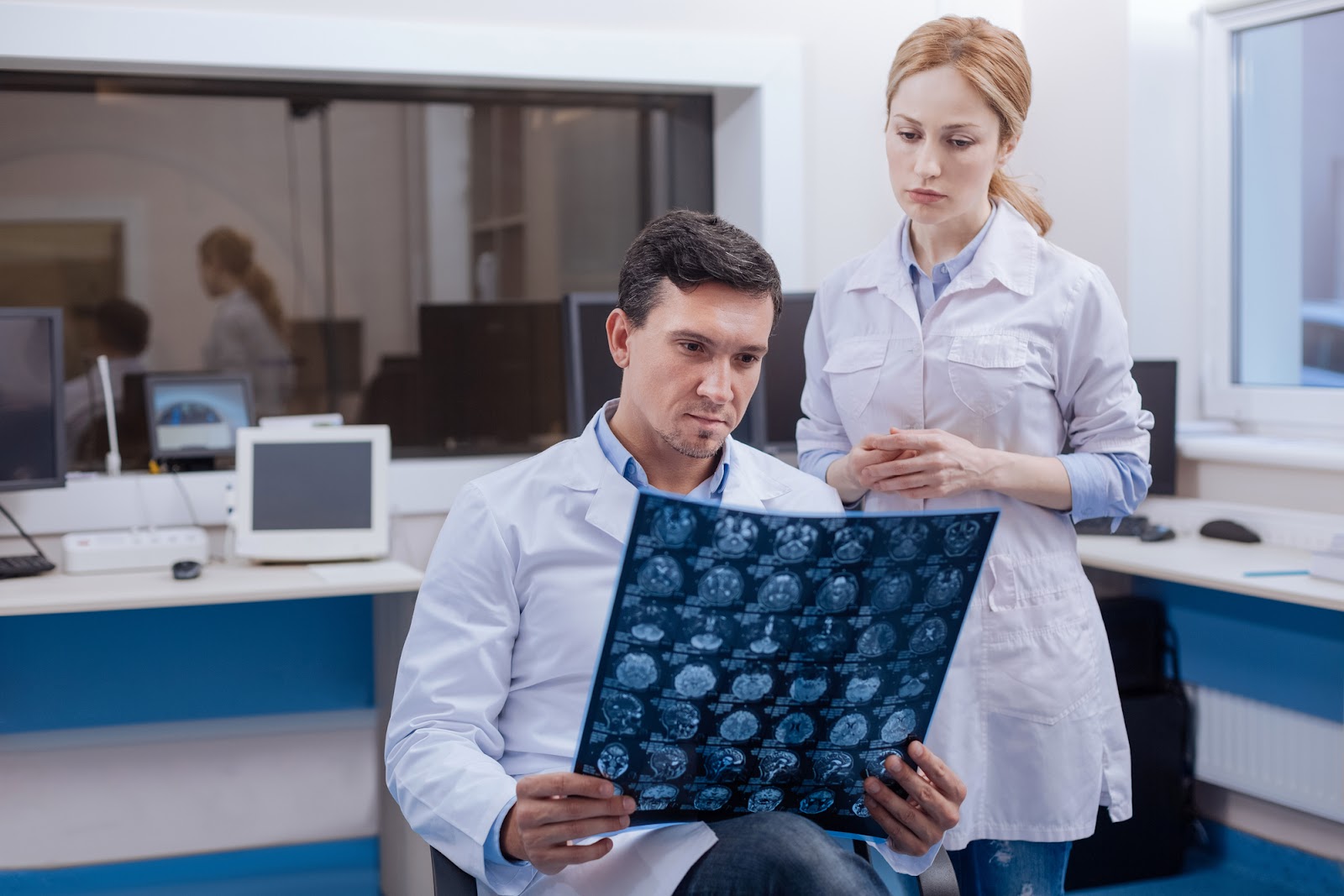
‍
Diagnostic Errors
‍
Medical malpractice is responsible for thousands of unnecessary deaths each year. In the United States, it accounts for an average of 40,000 to 80,000 deaths per year [5]. In Germany, medical malpractice accounts for up to 19,000 deaths per year [12]. Many of these malpractice errors stem from mistakes during diagnostics. While integrating AI tools could improve the accuracy of diagnostics, it’s not a foolproof system.Â
‍
These shortcomings were brought to light after two major studies were conducted to assess recently developed predictive AI tools in healthcare. Laure Wynants and her colleagues assessed 232 different algorithms for diagnosing patients and predicting the severity of their disease—none of which were found fit for clinical use [7].Â
‍
Similar results were found for the AI tools built to help diagnose COVID-19 and predict patient risk. A scientific review carried out by Derek Driggs and his colleagues examined 415 different machine learning models, primarily those revolving around COVID-19 diagnosis and predictions. Again, he found that none were fit for clinical use [7]. Some tools developed for COVID-19 even created inequality and bias in relation to data science due to a lack of sampling from certain groups—such as minorities or individuals with low socioeconomic status [6].According to researchers, this could lead to “biased research and policies that exacerbate pre-existing inequalities” [6].Â
‍
Although these reviews determined that AI tools were not ready for clinical use, both Wynants and Driggs believe that AI has the potential to help the healthcare industry. It just needs to be built and tested the right way.Â
‍
Roadblocks with Ethics and Regulation
‍
When doctors make a mistake or fail to provide the standard level of care, they can be sued for medical malpractice. Healthcare-related mistakes can be life or death, but who’s held responsible if the mistake occurs due to AI processing? This poses a question of ethics and regulation.Â
‍
Regulations put in place by privacy laws require transparency, which can impact the success of AI development across nations. Since each country or region needs to uphold specific regulations, it’s difficult to aggregate data without crossing an ethical (and sometimes illegal) line. Hospitals also often sign nondisclosure agreements with medical AI vendors, making them unable to discuss the algorithms or software they’re using [6]. This further complicates regulatory issues.Â
‍
In an effort to increase transparency, the World Health Organization recently released the Ethics and Governance of Artificial Intelligence for Health. It aims to identify ethical challenges and risks and outlines six consensus principles to ensure that AI works to the public benefit of all countries. It also includes recommendations for AI governance and accountability.Â
‍

‍
Black-Box Models of Processing
‍
The more data AI processes, the more complicated the algorithm becomes. The more complicated (or thorough) the algorithm gets, the better the outcome. So, more data = better outcomes. However, the more complex this process gets, the harder it is for healthcare workers to understand how or why AI tools got their results. This makes it difficult to determine the next steps.Â
‍
AI tools work in a “black box,” so there’s often a major lack of transparency, especially when deep learning models are used. Many healthcare professionals have no idea what’s being measured or how. This begs the question of effectiveness and accuracy. The black box problem is the primary reason people are hesitant to trust and accept the use of AI in healthcare.
Â
Explainable AI (XAI) was created to solve this issue. XAI methods show justification for how they came to a particular solution [2]. However, additional development is still needed to fully overcome the transparency problem.Â
‍
Privacy and Data Ownership ProblemsÂ
‍
The Health Insurance Portability and Accountability Act (HIPPA) was created with four primary objectives in mind. These objectives include:
‍
- “Assure health insurance portability by eliminating job-lock due to pre-existing medical conditions
- Reduce healthcare fraud and abuse
- Enforce standards for health information
- Guarantee security and privacy of health information” [9]
‍
There are also several stringent privacy laws in place, especially for healthcare data collected in European countries. The General Data Protection Regulation (GDPR) safeguards personal data, making it extraordinarily difficult, if not impossible, to share for use in AI development. Some organizations have even been sued for allegedly failing to uphold certain privacy standards.Â
‍
AI technology needs to be safeguarded and secured to uphold the privacy of patient data. However, AI data also needs to follow patients over the course of a long-term care program. This is necessary to better understand risk factors, medical conditions, symptoms, and long-term effects. This longitudinal approach to research requires ongoing engagement, which is difficult with privacy regulations. It gets even more complicated if the patient moves from one location (or country) to another.Â
‍

‍
Potential Future Applications of AI in Healthcare
‍
If researchers can overcome the challenges outlined above, AI tools could revolutionize the healthcare sector. They could help doctors identify patients who are at risk for developing chronic diseases or serious complications, improve the overall diagnostic process, and create a network of shared expertise available to doctors across the world. This could save lives, decrease the cost of medical care, and provide a better overall patient experience.
Â
Increased Accessibility, Decreased Cost
‍
Certain AI tools could aggregate and analyze millions of patients’ data. Other tools could analyze advice or experience from hundreds of thousands of physicians across the world. When combined, these tools could revolutionize how diagnoses are made and treatments are administered.Â
‍
It’s unlikely that AI tools will ever completely replace doctors, but they could help medical professionals excel in otherwise inaccessible areas of care. AI tools could help extend the reach and capabilities of doctors, thus increasing their ability to care for millions of patients. Medical AI can also help reduce the cost of health care.
‍
Administrative Tasks
‍
One of the most likely applications of AI technology in the healthcare industry is administrative or mundane tasks. AI could be used to capture live speech and dictate important information into a standardized note format. The notes could then be uploaded to databases. This would help doctors focus more on their patients and less on taking notes.Â
‍
Medical AI has the potential to increase doctor focus and individual cases, which can help reduce medical errors and improve patient care. Using information gathered during meetings, augmented intelligence devices would be most beneficial when used collaboratively. AI is unlikely to ever replace doctors. Instead, it would complement and augment them using unique strengths.Â
‍

‍
Monitoring of High-Risk Patients and Symptom Prediction
‍
Faster diagnostic processing improves patient care and comfort. It also allows for ongoing identification and monitoring of high-risk patients. VitalEye is an AI solution already in use. Through computer vision technology, breathing can be quickly detected and monitored, thus reducing the amount of patient prep time to under one minute [8]. Wearable devices can further monitor patients’ health remotely.Â
‍
Other systems could use patients’ medical documents and diagnostic results to help predict mortality, prolonged hospital care, or the risk of needing intensive care [10]. Wearable devices can improve early symptom prediction, which could help assess the risk of complications occurring. This could be particularly helpful for patients with heart disease or undergoing stroke rehabilitation. Wearable devices can also be used to help cryonics companies get notified when a member is in critical condition or if their heartbeat stops. This could help result in a higher quality cryopreservation.
‍
Infection Detection and Diagnostics
‍
Strategic AI tools could further improve the process of diagnostic evaluation or infection detection. Deep learning models could analyze higher volumes of data, imaging, and patient scans to help provide insight into potential diagnoses. Networking systems can work with various computer tomography to improve infection detection and diagnostics of diseases.Â
‍
Although challenges still exist, medical AI does have the potential to help expedite the diagnostic process. This is already becoming a reality. Consider the team of researchers at Philips and the University Medical Center of Leiden (LUMC). They developed a deep learning model that allowed MRI imaging to be performed eight times faster than current standards [8].Â
‍
Many real-world applications of this are currently being tested. For example, a new study shows that by combining AI with advanced imaging technology, brain tumors can be diagnosed in fewer than three minutes during surgery [15].Â
‍
Researchers at Tulane University also discovered that “AI can accurately detect and diagnose colorectal cancer… as well or better than pathologists” [13]. This detection can be done earlier and with higher rates of accuracy.Â
‍
Applications extend to other types of cancers as well. A study led by New York University researchers found that AI tools “increased radiologist’s ability to accurately identify breast cancer by 37%” [14]. This improvement in detection was also paired with a decrease in tissue necessary for sampling.Â
‍
Cryopreservation
‍
Artificial intelligence could also help improve the field of cryopreservation. Cryopreservation preserves cells and tissues by lowering core temperatures to sub-freezing levels without ice formation. However, there are currently several challenges to this process, especially in regard to cellular damage and viability after rewarming.Â
‍
AI could help identify which cryoprotective agents to use based on biological material and storage conditions. Technological advancements and AI may also allow for automation during the use of liquid nitrogen, thus improving overall safety within labs [16]. Companies like Future Fertility are already using AI to improve predictions on successful fertilization from cryopreserved eggs.
‍
‍
Drug Development and AnalyzationÂ
‍
Finally, overcoming current challenges facing AI in healthcare could help expedite drug development, especially during emergencies (i.e., global pandemics). AI tools could decrease the amount of time required to discover, develop, and analyze new drugs or treatments. This would allow for expedited use throughout the population and could potentially save millions of lives.Â
‍

Conclusion
‍
While prospective applications of AI in healthcare are vast, the challenges need to be addressed before any progress can be made. AI used within the medical field must adhere to a certain ethical standard, protect patient identity and data, and be standardized to optimize interoperability application. Once these challenges are overcome, the healthcare industry could be revolutionized.
‍
Who knows, AI tools may even help progress the development of cryopreservation technology and current cryopreservation applications more than we could ever imagine. In the meantime, if you have any questions about Biostasis, feel free to schedule a call with us. And, if you feel now ready to join our community, sign up here!
‍
‍
‍
‍
‍
References
[1] Asar, A. (2022, April 21). AI In Healthcare Presents Unique Challenges And Amazing Opportunities. Forbes. https://www.forbes.com/sites/forbestechcouncil/2021/11/22/ai-in-healthcare-presents-unique-challenges-and-amazing-opportunities/?sh=444832bb107bÂ
[2] Dilmegani, C. (2022, July 4). Top 4 Challenges of AI in Healthcare & How to Overcome Them. AIMultiple. https://research.aimultiple.com/challenges-of-ai-in-healthcare/Â
[3] Anderson, J. G. (2017). IOS Press Ebooks - Your Health Care May Kill You: Medical Errors. Https://Pubmed.Ncbi.Nlm.Nih.Gov/28186008/Â
[4] Watari, T. (2021, September 15). Malpractice Claims of Internal Medicine Involving Diagnostic and System Errors in Japan. 2021 by The Japanese Society of Internal Medicine. https://www.jstage.jst.go.jp/article/internalmedicine/60/18/60_6652-20/_articleÂ
[5] Renfrow, J. (2019, July 11). 1 in 3 misdiagnoses results in serious injury or death: study. Fierce Healthcare. https://www.fiercehealthcare.com/hospitals-health-systems/jhu-1-3-misdiagnoses-results-serious-injury-or-deathÂ
[6] The Alan Turing Institute. (2020). Data science and AI in the age of COVID-19. https://www.turing.ac.uk/sites/default/files/2021-06/data-science-and-ai-in-the-age-of-covid_full-report_2.pdfÂ
[7] Heaven, W. D. (2022, April 6). Hundreds of AI tools have been built to catch covid. None of them helped. MIT Technology Review. https://www.technologyreview.com/2021/07/30/1030329/machine-learning-ai-failed-covid-hospital-diagnosis-pandemic/Â
[8] Dickson, B. (2021, February 19). Meeting the challenges of AI in health care. TechTalks. https://bdtechtalks.com/2021/02/17/ai-healthcare-tina-manoharan-philips/Â
[9] Cutting Edge Document Destruction. (2011, November 24). Health Insurance Portability & Accountability Act (HIPAA) | Cutting Edge Document Destruction. https://cuttingedgedd.com/legislation/health-insurance-portability-accountability-act-hipaa/Â
[10] Mirin, K. (2021, December 2). Implementation of AI in Healthcare: Challenges and Potential. PostIndustria. https://postindustria.com/implementation-of-ai-in-healthcare-challenges-and-potential/Â
[11] Sullivan, T. (2019, April 1). Interoperability: 3 charts take the pulse of health data sharing today. Healthcare IT News. https://www.healthcareitnews.com/news/interoperability-3-charts-take-pulse-health-data-sharing-todayÂ
[12] Study: Hospital error kills 20,000 each year. (2014, January 21). The Local Germany. https://www.thelocal.de/20140121/more-die-from-hospital-mistakes-than-on-roads/
[13] McNemar, E. (2021, November 29). Top Opportunities for Artificial Intelligence to Improve Cancer Care. HealthITAnalytics. https://healthitanalytics.com/features/top-opportunities-for-artificial-intelligence-to-improve-cancer-careÂ
[14] McNemar, E. (2021a, September 28). Improving Breast Cancer Imaging with Artificial Intelligence. HealthITAnalytics. https://healthitanalytics.com/news/improving-breast-cancer-imaging-with-artificial-intelligenceÂ
[15] NCI Staff. (2020, February 12). Artificial Intelligence Expedites Brain Tumor Diagnosis. National Cancer Institute. https://www.cancer.gov/news-events/cancer-currents-blog/2020/artificial-intelligence-brain-tumor-diagnosis-surgeryÂ
[16] Parker, S. (2022, June 15). Artificial Intelligence is Essential to the Future of Cryopreservation. SmartData Collective. https://www.smartdatacollective.com/artificial-intelligence-is-essential-to-future-of-cryopreservation/Â