In today's rapidly changing world, the optimization of energy grid management has become more important than ever. One key tool that has emerged as a game-changer in this field is reinforcement learning. By harnessing the power of artificial intelligence, reinforcement learning offers a unique approach to improving energy efficiency and sustainability. In this article, we will explore the basics of reinforcement learning, its role in energy management, the process of implementing it in energy grid management, and the numerous benefits it brings. We will also take a glimpse into the future prospects of this exciting technology.
โ
Understanding Reinforcement Learning
โ
Before delving into its application in energy grid management, let's first grasp the basics of reinforcement learning. At its core, reinforcement learning is a type of machine learning where an agent learns to make decisions by interacting with its environment. It learns through a process of trial and error, receiving feedback in the form of rewards or punishments based on its actions.
โ
Reinforcement learning is inspired by the way humans and animals learn from their experiences. Just like a child learns to walk by taking small steps and adjusting their balance based on the feedback they receive, reinforcement learning algorithms learn to make decisions by taking actions and observing the outcomes.
โ
One of the key principles of reinforcement learning is the idea of maximizing cumulative rewards over time. The agent's goal is to learn a policy that maximizes the total reward it receives over the course of its interactions with the environment. This requires the agent to balance between exploration and exploitation.
โ
Exploration is the process of trying out different actions to gather information about the environment and learn which actions lead to higher rewards. Exploitation, on the other hand, involves taking actions that the agent believes will lead to the highest immediate reward based on its current knowledge.
โ
Reinforcement learning algorithms use various techniques to strike a balance between exploration and exploitation. One common approach is to use an epsilon-greedy policy, where the agent chooses the action with the highest estimated reward most of the time, but occasionally explores other actions with a small probability.
โ
Another important concept in reinforcement learning is the notion of learned policies. A policy is a mapping from states to actions, which tells the agent what action to take in a given state. Reinforcement learning algorithms learn policies by estimating the expected reward for each action in each state and selecting the action with the highest expected reward.
โ
By continuously exploring and exploiting the environment, reinforcement learning algorithms can discover optimal strategies to achieve specific goals. In the context of energy grid management, reinforcement learning can be used to optimize the scheduling of energy resources, balance supply and demand, and minimize costs.
โ
โ

โ
โ
The Role of Reinforcement Learning in Energy Management
โ
The need for optimization in energy grid management is pressing. Energy systems are becoming increasingly complex, with the integration of renewable energy sources, electric vehicles, and smart devices. To ensure efficient use of resources and reliable supply, it is imperative to employ intelligent solutions like reinforcement learning.
โ
One of the ways reinforcement learning enhances energy efficiency is through demand response. By analyzing real-time data, reinforcement learning algorithms can predict demand patterns and adjust energy generation and distribution accordingly. This dynamic optimization minimizes wastage and reduces reliance on non-renewable resources, contributing to a greener and more sustainable energy grid.
โ
In addition to demand response, reinforcement learning can also play a crucial role in energy storage management. With the increasing adoption of renewable energy sources such as solar and wind, the intermittent nature of these sources poses challenges in maintaining a stable energy supply. Reinforcement learning algorithms can optimize the charging and discharging of energy storage systems, ensuring efficient utilization of stored energy and minimizing grid instability.
โ
Furthermore, reinforcement learning can be applied to energy consumption optimization at the individual level. By analyzing historical data and user behavior, intelligent algorithms can identify patterns and make personalized recommendations to users for energy-efficient practices. This can include suggestions for adjusting thermostat settings, optimizing appliance usage, and adopting energy-saving habits. By empowering consumers with actionable insights, reinforcement learning contributes to a more energy-conscious society.
โ
Another area where reinforcement learning can make a significant impact is in the management of microgrids. Microgrids are localized energy systems that can operate independently or in conjunction with the main grid. They are particularly useful in remote areas or during emergencies when the main grid is unavailable. Reinforcement learning algorithms can optimize the operation of microgrids by balancing energy generation, storage, and consumption within the local network. This ensures reliable and efficient energy supply to the connected users, even in challenging conditions.
โ
Moreover, reinforcement learning can aid in the prediction and prevention of energy system failures. By continuously monitoring the performance of various components in the energy grid, intelligent algorithms can identify potential issues and take proactive measures to prevent failures. This predictive maintenance approach not only improves system reliability but also reduces downtime and maintenance costs.
โ
Furthermore, reinforcement learning can be utilized in the optimization of energy market operations. In deregulated energy markets, where multiple participants interact to buy and sell electricity, reinforcement learning algorithms can analyze market dynamics and make informed decisions regarding energy trading. By maximizing profits and minimizing risks, these algorithms contribute to the efficient functioning of energy markets.
โ
โ
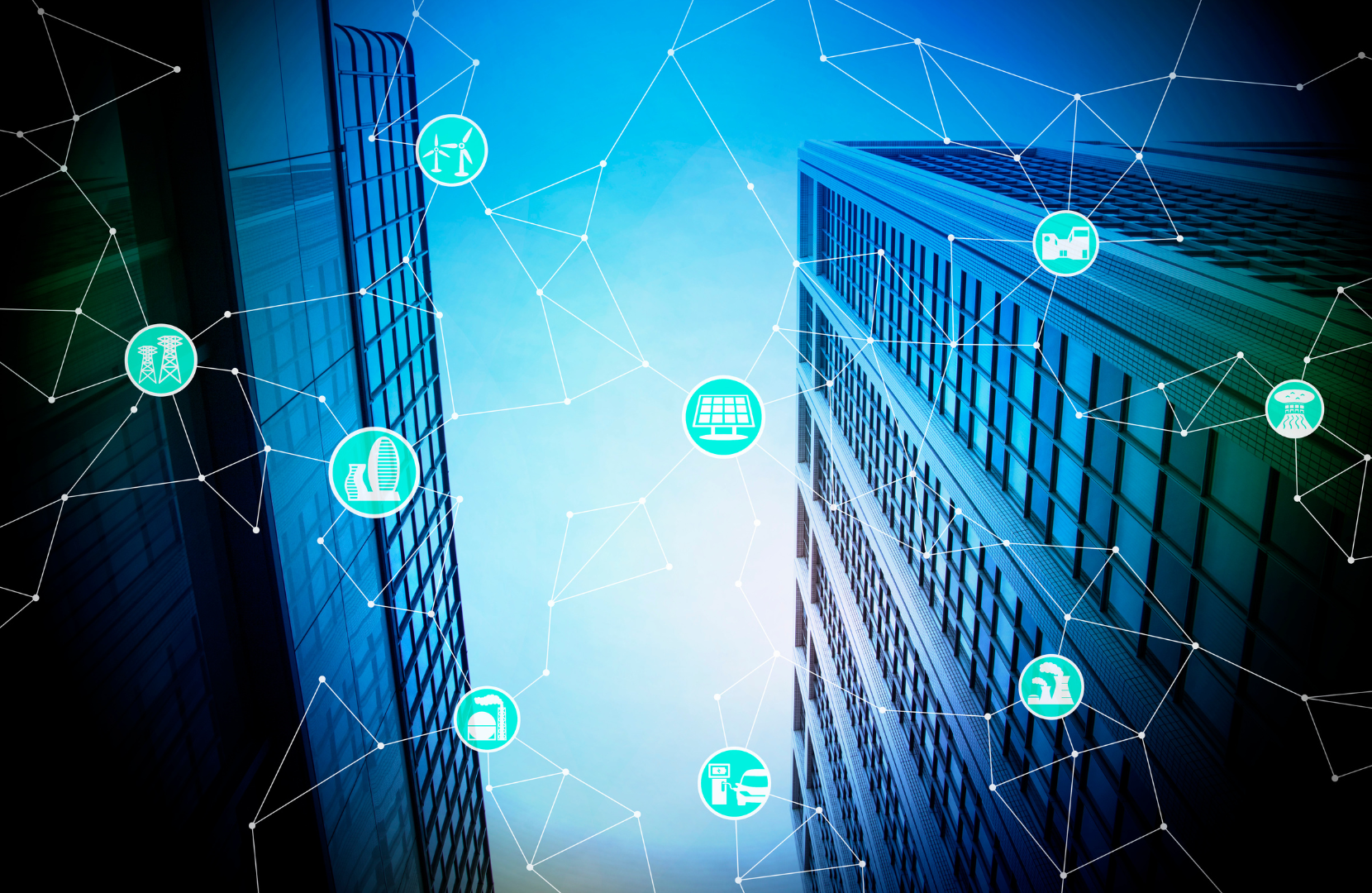
โ
โ
The Process of Reinforcement Learning in Energy Grid Management
โ
Data collection and analysis is a critical first step in implementing reinforcement learning in energy grid management. Accurate and reliable data is essential for training the algorithms and making informed decisions. With the advent of advanced sensors and IoT devices, large amounts of high-quality data can be collected in real-time.
โ
One of the key aspects of data collection in energy grid management is the use of smart meters. These devices are installed at various points in the grid and provide real-time information about energy consumption and generation. Smart meters enable the collection of granular data, allowing for a more detailed analysis of energy patterns and trends. This data includes information about peak demand periods, energy usage by different consumer segments, and fluctuations in renewable energy generation.
โ
Furthermore, the data collected from IoT devices, such as weather sensors and energy storage systems, adds another layer of information to the reinforcement learning process. Weather conditions play a significant role in energy grid management, as they impact both energy demand and generation. By integrating weather data into the algorithms, energy grid operators can make more accurate predictions and optimize their decision-making processes.
โ
Once the data is collected, it is fed into reinforcement learning algorithms, which use mathematical models to learn the optimal actions to maximize rewards. These algorithms consider variables such as energy demand, generation capacity, and weather conditions to make intelligent decisions. The learning process is ongoing, as the algorithms continuously update their policies based on new data and feedback.
โ
Reinforcement learning algorithms employ a trial-and-error approach to find the best strategies for energy grid management. They start with random actions and observe the outcomes to determine which actions lead to the highest rewards. Over time, the algorithms learn to associate certain actions with positive outcomes and adjust their policies accordingly.
โ
One of the challenges in reinforcement learning for energy grid management is the complexity of the system. The energy grid is a highly interconnected network with various components, including power plants, transmission lines, substations, and distribution networks. Each component has its own characteristics and constraints, making it challenging to develop a unified model for the entire system.
โ
To address this challenge, researchers are developing multi-agent reinforcement learning algorithms that can handle the complexity of the energy grid. These algorithms enable different agents to learn and interact with each other, simulating the decentralized decision-making process in the energy grid. By allowing agents to communicate and share information, these algorithms can optimize the overall performance of the grid and ensure a reliable and efficient energy supply.
โ
Benefits of Using Reinforcement Learning in Energy Grid Management
โ
The advantages of using reinforcement learning in energy grid management are manifold. Firstly, it leads to improved energy efficiency and sustainability. With better prediction and control of energy demand, wastage is minimized, leading to reduced environmental impact.
โ
Cost savings and economic benefits are another significant advantage. By optimizing energy usage, reinforcement learning algorithms can help reduce operational costs and increase revenue. This is particularly valuable in the current landscape of rising energy prices and the need for budget-conscious strategies.
โ
โ
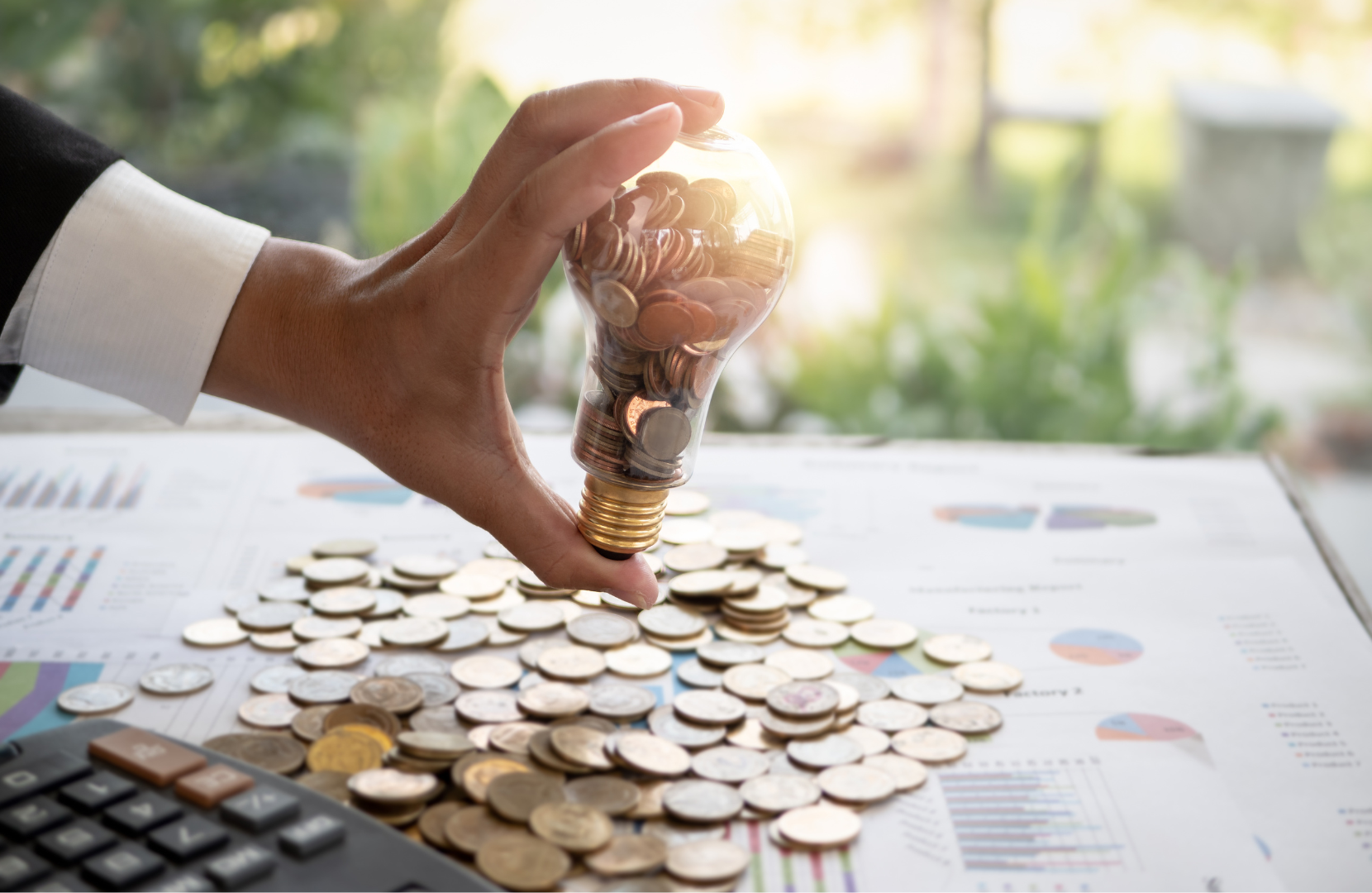
โ
โ
Future Prospects of Reinforcement Learning in Energy Management
โ
As we look to the future, the prospects of reinforcement learning in energy management are incredibly promising. The field is constantly evolving, with emerging trends and innovations driving advancements. For example, the integration of reinforcement learning with other AI techniques, such as deep learning, has the potential to unlock new levels of optimization and efficiency.
โ
However, challenges need to be addressed for the widespread adoption of reinforcement learning in energy grid management. Issues related to data privacy, security, and the interpretability of complex models must be carefully considered and resolved.
โ
Despite these challenges, it is clear that reinforcement learning has the potential to revolutionize energy grid management. By leveraging the power of artificial intelligence, we can create a more sustainable, efficient, and cost-effective energy future.