In the fast-paced world of artificial intelligence (AI), the need for reliable testing methods has become increasingly crucial. One such method that has gained significant attention is simulated reality testing. By replicating real-world scenarios within a controlled virtual environment, developers can assess the performance and capabilities of AI models. In this article, we will explore the concept of simulated reality, its role in AI development, the intersection of AI and simulated reality, the process of simulated reality testing, the challenges and solutions in this testing method, and finally, the future outlook of simulated reality testing in AI.
โ
Understanding Simulated Reality
โ
The concept of simulated reality revolves around creating an immersive virtual environment that mimics real-world situations. Through cutting-edge technologies, such as virtual reality (VR) and augmented reality (AR), developers can simulate various scenarios that an AI model might encounter in the real world. This allows them to train and evaluate AI models in a controlled, safe, and cost-effective manner.
โ
The Concept of Simulated Reality
โ
Simulated reality refers to a computer-generated environment that replicates reality to a convincing degree. By incorporating elements such as physics, graphics, and user interaction, developers strive to create a virtual world indistinguishable from the real one. Simulated reality testing leverages this concept by placing AI models in these virtual environments to assess their performance and capabilities.
โ
Imagine stepping into a simulated reality where you find yourself in the bustling streets of a city. As you walk down the virtual sidewalks, you can hear the sounds of cars passing by, people chatting, and birds chirping in the distance. The buildings around you stand tall and vibrant, with intricate details that make them look and feel real. You can interact with objects, open doors, and even have conversations with virtual characters who respond intelligently to your queries.
โ
The level of immersion in simulated reality is truly remarkable. Every step you take, every decision you make, and every interaction you have feels as if you are in a parallel universe. The developers behind simulated reality put immense effort into creating a seamless experience that engages all your senses, making it difficult to distinguish between the virtual and the real.
โ
โ
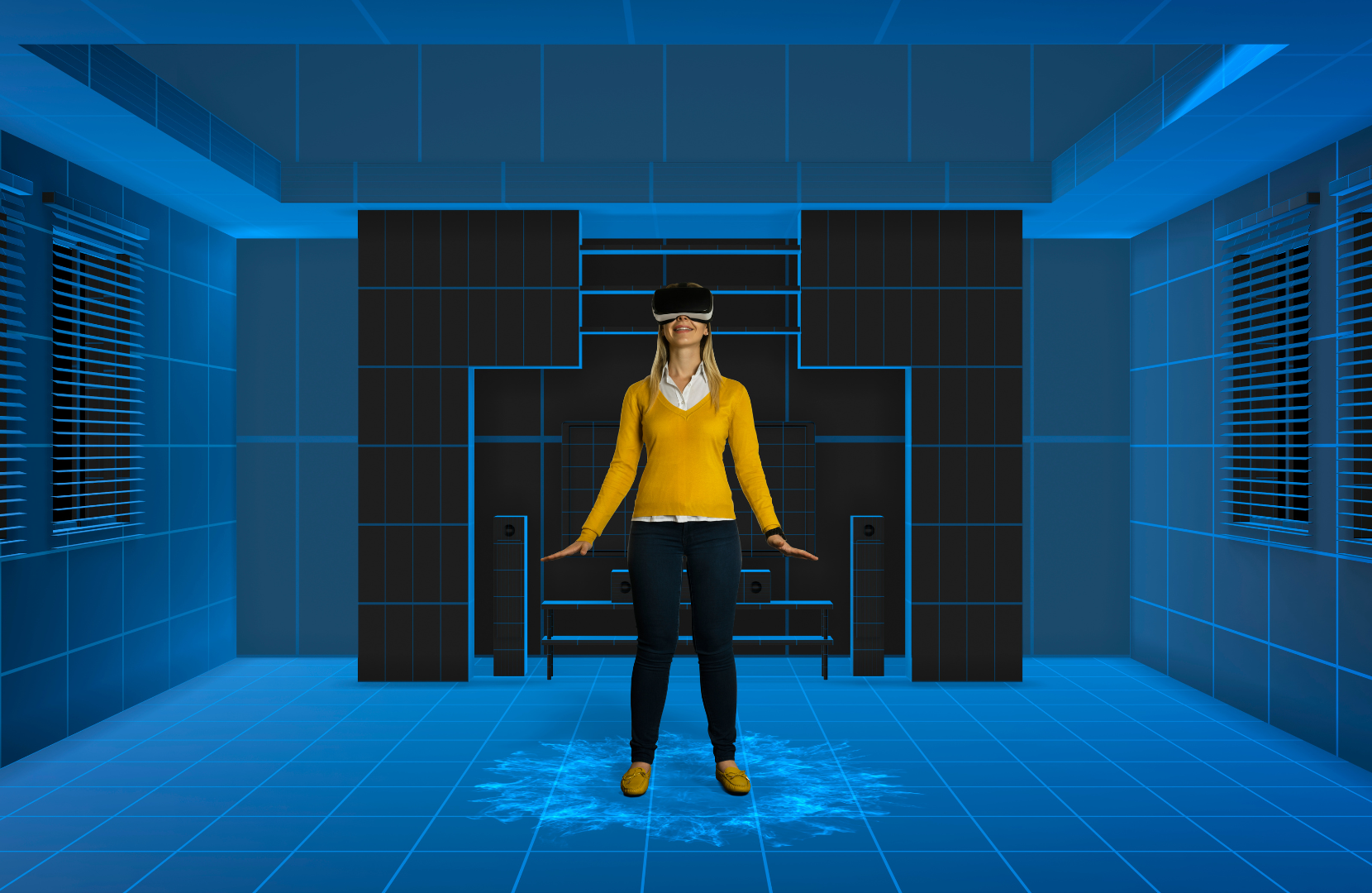
โ
โ
The Role of Simulated Reality in AI Development
โ
Simulated reality plays a critical role in the development of AI models. It allows developers to test and refine their models efficiently before deploying them into the real world. By subjecting AI models to a wide range of simulated scenarios, developers can identify potential weaknesses, make necessary adjustments, and enhance the models' learning capabilities.
โ
Let's delve deeper into how simulated reality aids in AI development. Imagine an AI model designed to navigate through complex environments, such as a self-driving car. Simulated reality provides a platform where developers can create virtual roads, traffic, and pedestrians to train the AI model. The AI model can learn to recognize traffic signs, anticipate pedestrian movements, and make split-second decisions without the risk of real-world accidents.
โ
Simulated reality also enables developers to introduce challenging scenarios that may be difficult to replicate in the real world. For instance, an AI model designed to assist in medical diagnosis can be trained in a simulated environment where it encounters a wide range of rare diseases and complex symptoms. This allows the model to learn and adapt to various medical conditions, improving its accuracy and reliability when deployed in real healthcare settings.
โ
Furthermore, simulated reality offers a cost-effective solution for AI development. Building physical prototypes or conducting large-scale real-world experiments can be expensive and time-consuming. Simulated environments eliminate these limitations by providing a virtual space where developers can iterate and experiment with their AI models without incurring significant costs. This accelerates the development process and enables faster innovation in the field of AI.
โ
โ
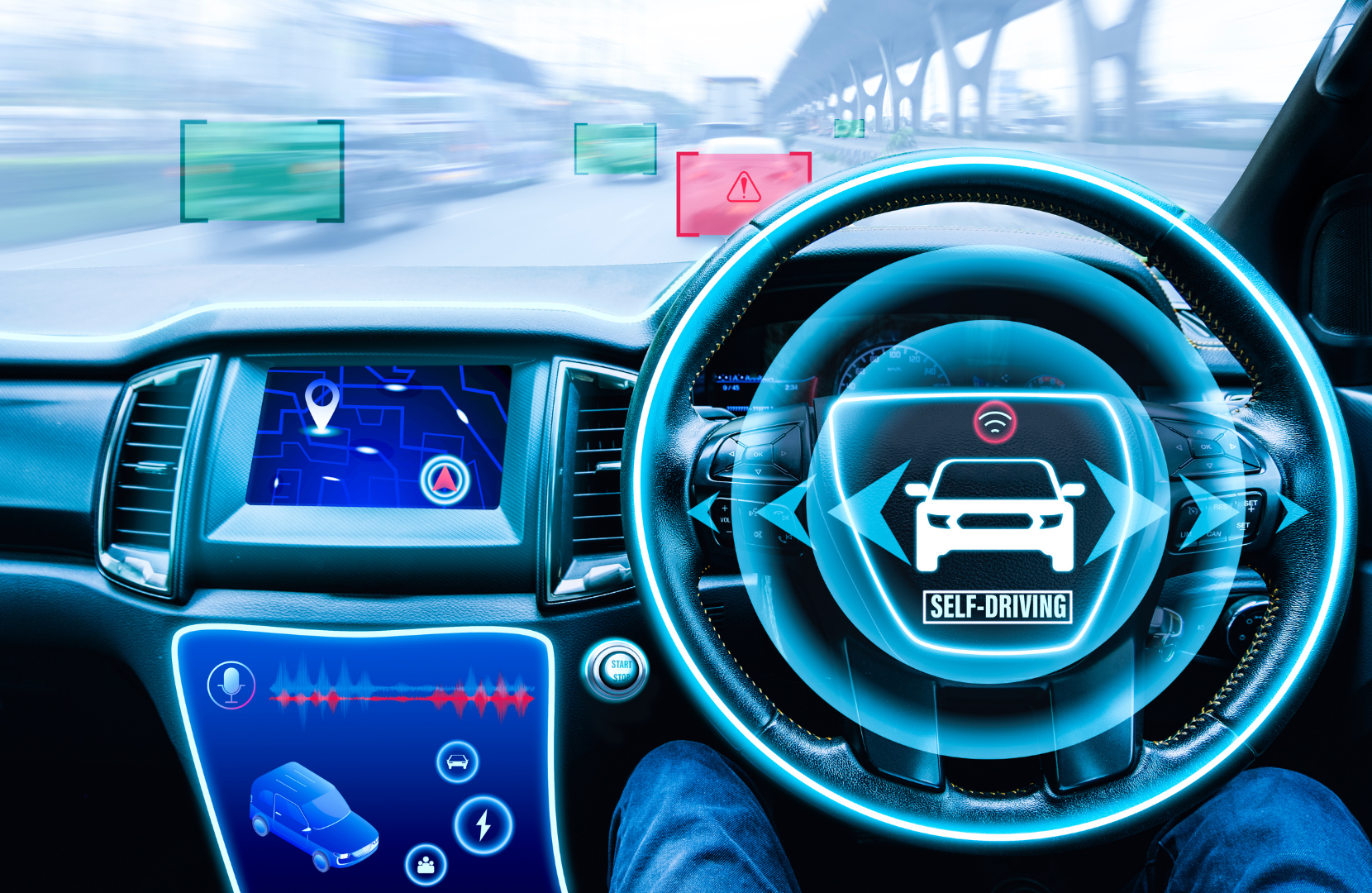
โ
โ
The Intersection of AI and Simulated Reality
โ
The intersection of AI and simulated reality creates a promising synergy that benefits both fields. AI models can interact with simulated reality environments, enabling them to gather invaluable data and provide accurate predictions for real-world scenarios.
โ
When AI models interact with simulated reality, they are able to navigate and interpret these virtual environments using a combination of sensory input and decision-making algorithms. These models are specifically designed to learn from the data they gather in these virtual environments, continuously improving their performance and decision-making abilities over time.
โ
One of the key benefits of using simulated reality in AI testing is the ability to create a controlled environment. Developers can isolate specific variables and thoroughly evaluate an AI model's performance without any external factors influencing the results. This controlled environment allows for a more accurate assessment of the model's capabilities and potential limitations.
โ
Furthermore, simulated reality eliminates the risks and costs associated with conducting tests in the real world. In the real world, there are numerous variables that are difficult to control, making it challenging to accurately assess an AI model's performance. Simulated reality provides a safe and cost-effective alternative, allowing developers to experiment freely and iterate quickly.
โ
By using simulated reality in AI testing, developers can also explore a wide range of scenarios and edge cases that may be difficult or even impossible to replicate in the real world. This enables them to uncover potential issues and vulnerabilities in the AI model's behavior, leading to more robust and reliable systems.
โ
Moreover, simulated reality offers the opportunity to gather large amounts of data in a short period of time. In the real world, data collection can be time-consuming and expensive. Simulated reality environments provide a way to generate vast amounts of data quickly, allowing AI models to learn and adapt at an accelerated pace.
โ
โ

โ
โ
The Process of Simulated Reality Testing
โ
Simulated reality testing involves a structured approach to ensure accurate and insightful results. This process includes preparing AI models for simulated reality testing and conducting tests following a set of predefined steps.
โ
Preparing AI Models for Simulated Reality Testing
โ
Prior to testing, AI models must be trained using available data sets and algorithms. This initial preparation stage ensures that the models have a foundation to perform well in simulated reality environments. The training phase also involves fine-tuning the models to adapt to the specific challenges they may encounter in the virtual world.
โ
Steps in Conducting Simulated Reality Tests
โ
Conducting simulated reality tests involves a series of steps to ensure accurate assessment and analysis of AI models. These steps include defining the objectives of the test, creating a realistic environment, running the tests with varied scenarios, collecting comprehensive data, and analyzing the results for further improvements.
โ
Challenges and Solutions in Simulated Reality Testing
โ
While simulated reality testing provides immense advantages, it also presents unique challenges that developers need to address. By identifying and overcoming these obstacles, developers can optimize the testing process and achieve more reliable results.
โ
Common Obstacles in Simulated Reality Testing
โ
One of the challenges in simulated reality testing is creating virtual environments that accurately reflect the complexities and nuances of the real world. Ensuring the realism of virtual scenarios, as well as accounting for unpredictable real-world elements, requires constant innovation and refinement.
โ
Innovative Solutions for Simulated Reality Testing
โ
Developers continuously explore innovative solutions to enhance the accuracy and authenticity of simulated reality testing. This includes using advanced physics engines, improving graphical fidelity, integrating real-time data feeds, and incorporating machine learning algorithms into the virtual environment to account for dynamic real-world factors.
โ
The Future of Simulated Reality Testing in AI
โ
As AI continues to evolve, so too will the practice of simulated reality testing. Looking ahead, several promising trends indicate the potential impact of advancements in AI and simulated reality testing.
โ
Predicted Trends in Simulated Reality Testing
โ
Experts predict a rise in the complexity and intricacy of simulated reality environments, enabling AI models to tackle more sophisticated challenges. Additionally, the integration of emerging technologies, such as augmented reality and mixed reality, is expected to further enhance the realism and applicability of simulated reality testing.
โ
The Impact of Advancements in AI on Simulated Reality Testing
โ
The continuous advancements in AI will significantly influence the future of simulated reality testing. AI models with enhanced learning capabilities will be able to adapt and respond more effectively to simulated scenarios, supporting the development of robust and reliable AI systems.
โ
Conclusion
โ
Simulated reality testing represents a valuable tool in the development and evaluation of AI models. By replicating real-world scenarios within virtual environments, developers can iteratively improve their models and enhance their performance. As AI and simulated reality continue to advance, the possibilities for testing and refining AI models in a safe and controlled environment will expand, leading to increasingly sophisticated AI systems that can tackle real-world challenges effectively.