In today's rapidly advancing technological landscape, artificial intelligence (AI) plays an increasingly significant role. Its potential to improve various industries, from healthcare to finance, is undeniable. However, like any other system, AI requires training to perform optimally. Drawing inspiration from expert humans, strategies for AI training are being developed and implemented to bridge the gap between machine learning and human expertise. In this article, we will delve into the concept of AI training, explore the role of expert humans in the process, discuss strategies employed for AI training, examine the challenges faced when integrating human expertise, and take a glimpse into the future of AI training.
โ
โ
Understanding the Concept of AI Training
โ
Before we dive into the intricacies of AI training, it's crucial to establish a clear understanding of artificial intelligence itself. AI refers to the design and development of computer systems that can simulate human intelligence, allowing them to analyze data, make decisions, and perform tasks without explicit programming.
โ
Artificial intelligence has revolutionized various industries, from healthcare and finance to transportation and entertainment. It has enabled machines to perform complex tasks that were once exclusive to human capabilities. With AI, we can now automate processes, analyze vast amounts of data, and even create intelligent virtual assistants.
โ
However, for AI systems to reach their full potential, they need to undergo a process called training. Training is a vital component of AI development. Through training, AI systems can acquire knowledge and skills, refine their algorithms, and continuously improve their performance based on data analysis.
โ
Training an AI system involves exposing it to large amounts of data and providing it with the necessary tools to learn from that data. This process is similar to how humans learn from experience. The more data an AI system is exposed to, the better it becomes at recognizing patterns, making predictions, and making informed decisions.
โ
During the training process, AI systems use algorithms to analyze the data and identify patterns and correlations. These algorithms are designed to adapt and adjust based on the input they receive. As the AI system continues to learn and analyze data, it becomes more accurate and efficient in its decision-making process.
โ
One of the key advantages of AI training is its ability to handle complex and unstructured data. Traditional programming methods often struggle with unstructured data, such as images, videos, and natural language. AI training allows systems to process and understand this type of data, opening up new possibilities for applications in various fields.
โ
Moreover, AI training enables systems to adapt to changing environments and circumstances. By continuously analyzing new data, AI systems can update their knowledge and adjust their algorithms to make more accurate predictions and decisions. This adaptability is crucial in dynamic industries where conditions and requirements change frequently.
โ
In conclusion, training is an essential step in the development of AI systems. It allows these systems to acquire knowledge, refine their algorithms, and continuously improve their performance. With proper training, AI systems can simulate human intelligence, analyze complex data, and make informed decisions, revolutionizing industries and enhancing our daily lives.
โ
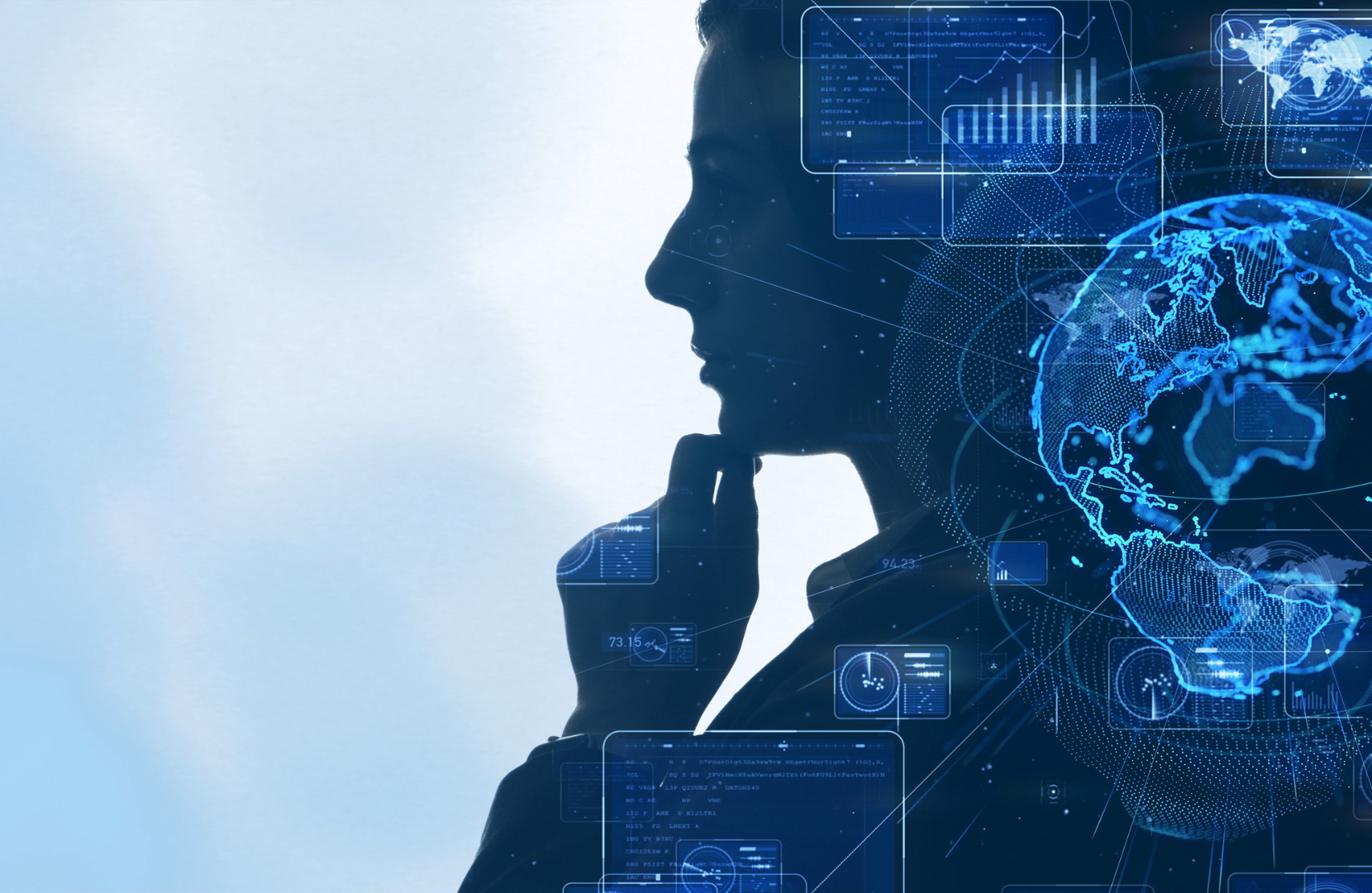
โ
The Role of Expert Humans in AI Training
โ
While AI technology is undoubtedly impressive, the involvement of expert humans remains invaluable during the training process. Identifying these expert humans, who possess extensive domain knowledge, is crucial for developing effective training strategies.
โ
โ
Identifying Expert Humans
โ
Expert humans are individuals who have accumulated significant expertise in a particular domain. These experts possess deep insights, understanding, and intuition based on their extensive experience and knowledge.
โ
When it comes to identifying expert humans, there are various methods that can be employed. One approach is to look for individuals who have a proven track record of success in the field. These experts may have published groundbreaking research papers, received prestigious awards, or made significant contributions to the industry. Another approach is to seek recommendations from peers and colleagues who are familiar with the domain. Their insights can help identify individuals who have a reputation for being knowledgeable and skilled in the field.
โ
โ
The Value of Human Expertise in AI
โ
Human expertise brings a unique perspective and critical thinking to AI training. Their insights help identify relevant data, develop efficient algorithms, and ensure that the AI system aligns with real-world scenarios.
โ
One of the key advantages of involving expert humans in AI training is their ability to provide context. While AI algorithms excel at processing large amounts of data and identifying patterns, they often lack the ability to understand the underlying meaning and context. Expert humans, on the other hand, can provide valuable context that helps the AI system make more informed decisions.
โ
Furthermore, human experts can help address the issue of bias in AI systems. AI algorithms are trained on data, and if the training data is biased, the AI system will also exhibit bias in its decision-making. Human experts can play a crucial role in identifying and mitigating bias by carefully curating the training data and ensuring that it is representative and diverse.
โ
In addition to providing context and addressing bias, human expertise is also essential in handling complex and ambiguous situations. AI systems often struggle when faced with scenarios that require nuanced understanding or subjective judgment. Expert humans can provide guidance and insights in such situations, helping the AI system navigate through the complexities and make more accurate decisions.
โ
Overall, the involvement of expert humans in AI training is vital for the development of robust and effective AI systems. Their domain knowledge, insights, and critical thinking complement the capabilities of AI technology, ensuring that the resulting systems are not only intelligent but also reliable, fair, and aligned with real-world needs.
โ
โ
Strategies for AI Training
โ
Developing and implementing effective strategies for AI training is vital to unlock the full potential of machine learning. By combining the expertise of humans and the processing power of AI systems, organizations can achieve remarkable results.
โ
โ
Strategy Development and Implementation
โ
Developing an AI training strategy involves defining the objectives, determining the training data, and selecting appropriate algorithms. It is crucial to strike a balance between supervised and unsupervised learning, depending on the desired outcomes.
โ
When it comes to strategy development, organizations need to consider the specific problem they are trying to solve with AI. For instance, in the healthcare industry, AI can be used to analyze medical images and detect diseases. In this case, the training data would consist of a large number of labeled medical images, along with corresponding diagnoses. The algorithm chosen would need to be capable of accurately classifying the images and providing accurate diagnoses.
โ
Another important aspect of strategy development is determining the availability and quality of training data. In some cases, organizations may need to collect and label their own data, while in others, pre-existing datasets can be used. The quality of the data is also crucial, as AI models are only as good as the data they are trained on. Organizations need to ensure that the training data is representative of the real-world scenarios the AI system will encounter.
โ
โ
Commonly Used Strategies in AI Training
โ
Various strategies are employed in AI training. Supervised learning utilizes labeled data to train AI models, while unsupervised learning aims to discover patterns from unlabeled data. Reinforcement learning involves rewarding or penalizing AI systems based on their actions, encouraging them to learn from their mistakes and refine their decision-making abilities.
โ
Supervised learning is often used when there is a clear understanding of the desired outcome and labeled data is readily available. For example, in the field of natural language processing, supervised learning can be used to train AI models to classify text into different categories, such as sentiment analysis or topic classification. The labeled data would consist of a large number of text samples, each labeled with the corresponding sentiment or topic.
โ
Unsupervised learning, on the other hand, is used when the desired outcome is not known or when labeled data is scarce. This approach allows AI models to discover patterns and relationships in the data without any prior knowledge. An example of unsupervised learning is clustering, where AI models group similar data points together based on their features. This can be useful in customer segmentation or anomaly detection.
โ
Reinforcement learning is a strategy that involves training AI models through a system of rewards and penalties. The AI system learns by interacting with an environment and receiving feedback based on its actions. This approach is often used in robotics or game playing, where the AI system needs to learn how to make decisions and improve its performance over time.
โ
In conclusion, developing and implementing effective strategies for AI training is crucial for organizations to harness the power of machine learning. By carefully considering the objectives, training data, and algorithms, organizations can train AI models that can make accurate predictions, discover hidden patterns, and make informed decisions.
โ
.png)
โ
Bridging the Gap Between AI and Human Expertise
โ
Integrating human expertise into AI systems presents unique challenges and opportunities. While it may seem daunting, finding effective solutions is crucial to unleash the true potential of AI.
โ
โ
Challenges in Integrating Human Expertise into AI
โ
One of the primary challenges when integrating human expertise into AI is capturing the tacit knowledge possessed by experts. Tacit knowledge refers to skills and insights that are difficult to articulate explicitly. Translating this knowledge into algorithms that can be understood by AI systems is a complex task.
โ
โ
Solutions and Innovations
โ
To address these challenges, researchers are exploring innovative methods such as natural language processing and deep learning techniques. These advancements aim to improve the ability of AI systems to understand and integrate human expertise.
โ
โ
The Future of AI Training with Human Expertise
โ
As AI continues to evolve, so does the role of human expertise in training these systems. Let's take a glimpse into the future and explore the predicted trends and developments.
โ
โ
Predicted Trends and Developments
โ
In the future, AI training will likely become more sophisticated and specialized. AI systems will be trained by combining human expertise with vast amounts of data, allowing them to make more accurate predictions and solve complex problems across various industries.
โ
โ
The Impact on Various Industries
โ
The integration of AI training and human expertise is poised to revolutionize numerous industries. From healthcare diagnostics that leverage the knowledge of expert physicians to financial analysis that combines economic expertise with AI algorithms, the possibilities for growth and improvement are virtually limitless.
โ
In conclusion, training AI with strategies inspired by expert humans holds immense potential. By combining the power of AI systems with the deep insights of human expertise, we can unlock new possibilities in various industries. As technology continues to advance and researchers push the boundaries of AI training, we can expect transformative developments that will reshape the way we interact with AI systems. Embracing this collaboration between humans and machines will undoubtedly pave the way for a future where AI can augment and enhance human capabilities like never before.