Deep learning models are increasingly being used to make important decisions in various industries such as healthcare, finance, and criminal justice. However, studies have shown that these models are not as neutral as we typically assume them to be. Instead, they are often found to be systematically oppressive. In this article, we will discuss the concept of "neutral" deep learning models and explore how they contribute to oppression. We will also examine the sources of bias in AI systems and the real-world consequences of systematic oppression. Finally, we will offer strategies to mitigate bias in deep learning models and encourage transparency in AI development.
Understanding the Concept of "Neutral" Deep Learning Models
Deep learning models are a subset of artificial intelligence that can learn and perform tasks without being explicitly programmed to do so. The key advantage of these models is their ability to learn from large datasets, which allows them to make predictions and decisions with a high level of accuracy.
However, deep learning models are not infallible. They can be biased in their decision-making processes, leading to unfair or problematic outcomes. The problem arises when we assume that these models are “neutral,” free of any pre-existing biases or prejudices, and therefore objective. This assumption can lead us to overlook the ways in which deep learning models systematically oppress certain groups of people.
Defining Deep Learning Models
Deep learning models start with a neural network that’s trained using large amounts of data. This data is typically used to identify patterns that will help the model to make accurate predictions about new data that it hasn’t seen before. Deep learning models can be used in a wide range of applications, from self-driving cars to personal digital assistants.
For example, a deep learning model can be trained to recognize images of cats. The model is fed thousands of images of cats and learns to identify common features, such as pointy ears and whiskers. Once the model has been trained, it can accurately identify images of cats that it has never seen before.
Another example of a deep learning model is a natural language processing (NLP) model. This type of model can be trained to understand human language and respond to requests in a conversational manner. NLP models are used in personal digital assistants, such as Siri and Alexa, to help users complete tasks and answer questions.

The Illusion of Neutrality in AI Systems
The idea that deep learning models are neutral is an illusion that is perpetuated by the lack of transparency in AI systems. Many deep learning models are “black boxes,” meaning that we can’t see what’s happening inside the model. This lack of transparency makes it difficult to identify sources of bias and mitigate them.
For example, let’s say a deep learning model is used to predict which job candidates are most likely to be successful in a particular role. The model is trained on historical data, which includes information about the job performance of previous employees. However, if the historical data is biased, the model will also be biased. If the historical data is biased against women or people of color, for example, the model will be biased against these groups as well.
It’s important to note that bias in deep learning models is not always intentional. In many cases, the biases are unintentional and stem from the data that’s used to train the model. However, it’s still important to be aware of these biases and take steps to mitigate them.
One way to mitigate bias in deep learning models is to use diverse datasets that represent a wide range of perspectives. This can help to ensure that the model is not biased towards any particular group. Additionally, it’s important to regularly test and evaluate deep learning models to identify any sources of bias and make adjustments as necessary.
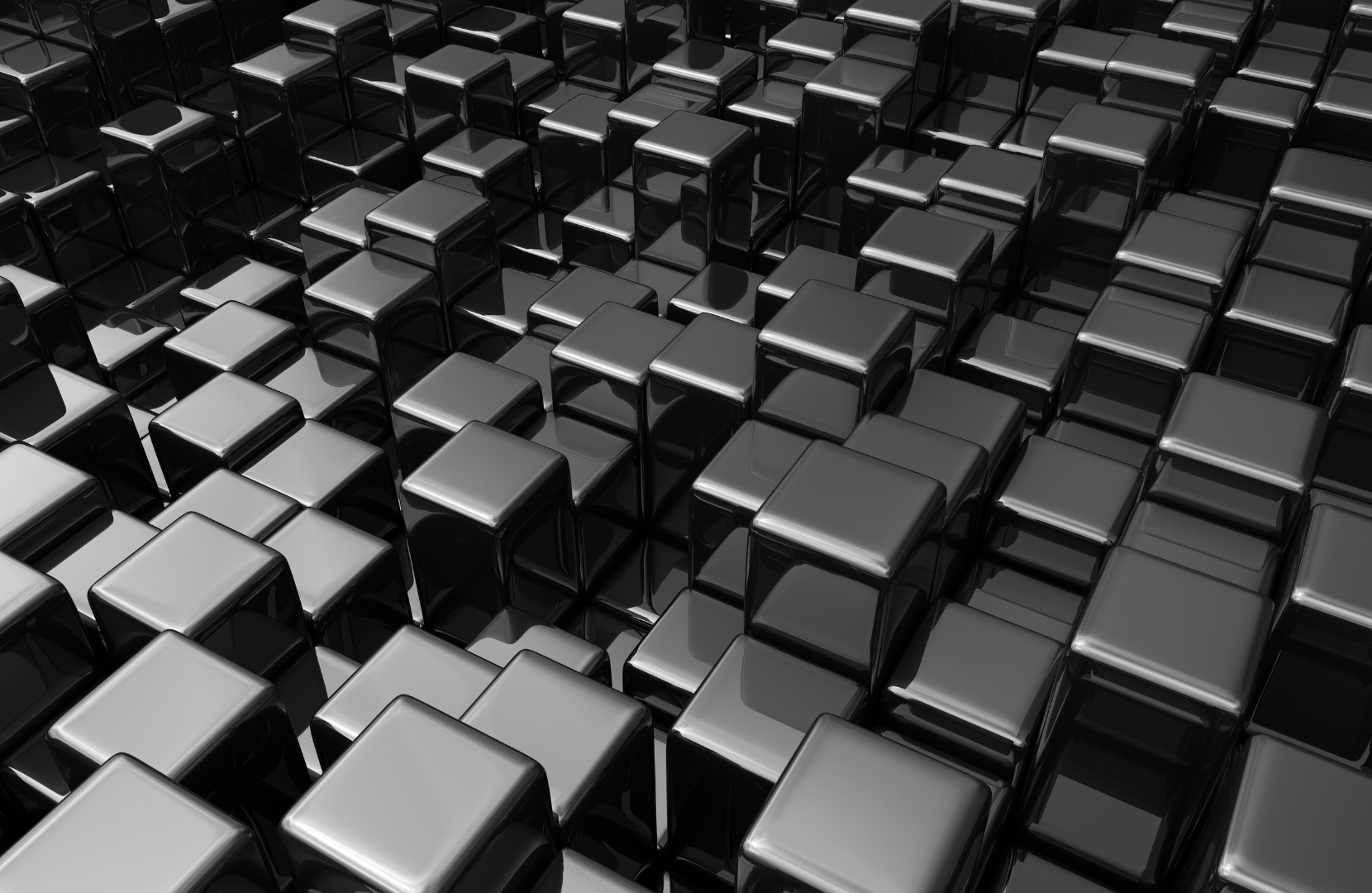
The Role of Bias in Deep Learning Models
Bias is a complex issue that can have significant implications in the development and deployment of deep learning models. In the context of artificial intelligence (AI), bias can be defined as any systemic or unconscious preference or prejudice that affects the way a model makes decisions or interprets data. Bias can arise from a variety of sources, including the data used to train the model, the assumptions made by the model's creators, and the algorithm used to make decisions.
Sources of Bias in AI Systems
One of the most common sources of bias in deep learning models is the dataset used to train the model. If the dataset is not diverse enough, it may not accurately represent all the groups that the model will encounter in the real world. For example, if a facial recognition model is trained only on white faces, it may struggle to recognize faces of other races, leading to discriminatory outcomes. Similarly, if a job applicant screening model is trained on data that skews towards certain demographic groups, it may inadvertently reject candidates from other groups.
Another source of bias in AI systems is the assumptions made by the model's creators. If the creators of a model have unconscious biases, those biases can be reflected in the model's decisions. For example, if the creators of a hiring model assume that men are better suited for certain types of jobs, the model may be biased against female candidates.
The algorithm used to make decisions can also introduce bias into deep learning models. Some algorithms are inherently biased, either because of the way they are designed or because of the data they use to make decisions. For example, an algorithm that is designed to identify high-risk patients may be biased against patients from certain demographic groups if the data used to train the algorithm is not representative of the population as a whole.
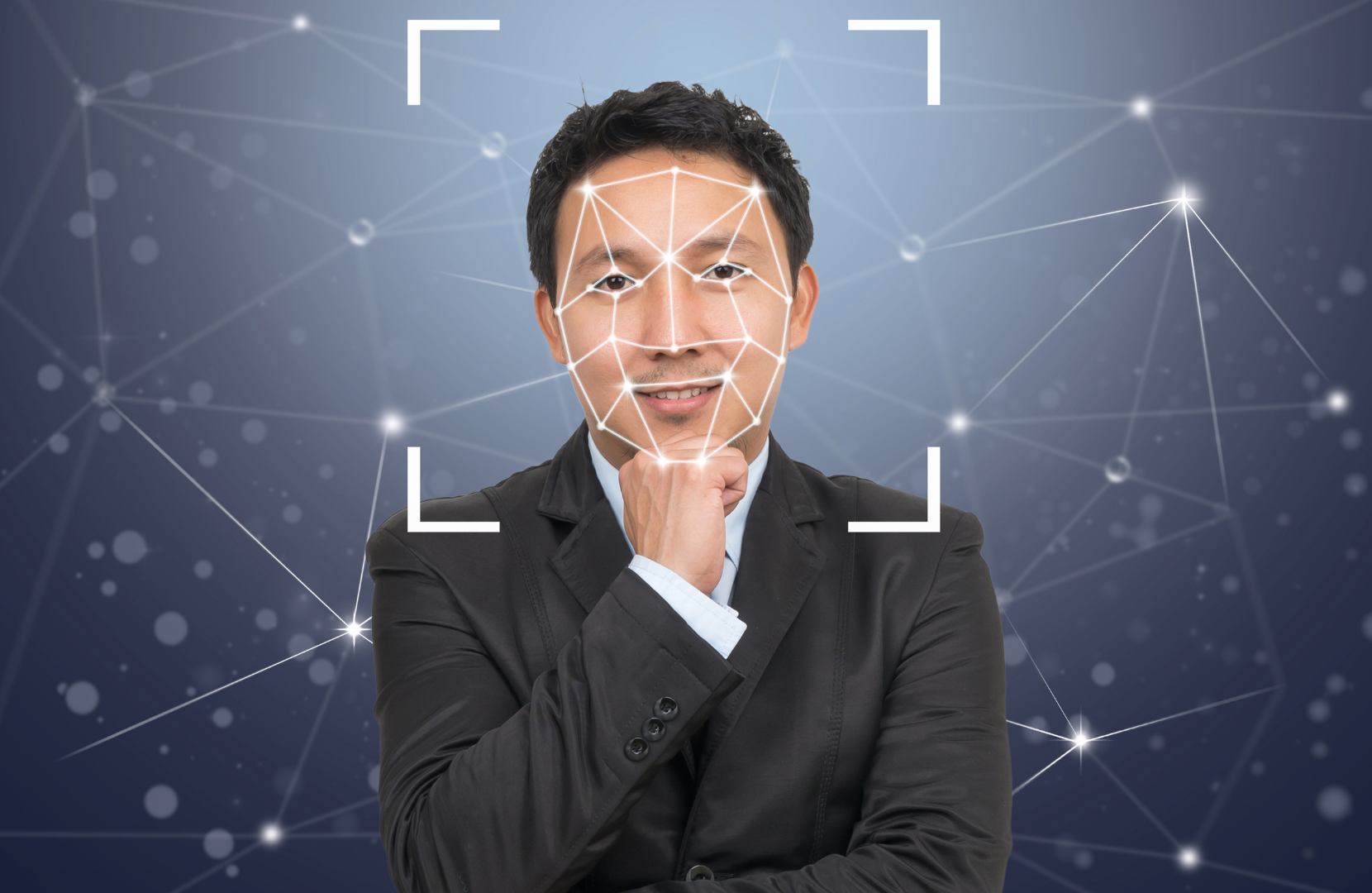
How Bias Affects Model Outcomes
Bias in deep learning models can have serious consequences. In some cases, it can reinforce harmful stereotypes and perpetuate inequality. For example, a facial recognition model that consistently identifies black people as criminals may reinforce existing stereotypes about criminality and racial profiling. In other cases, it can limit opportunities for marginalized groups. For example, a job screening model that is biased against candidates from certain demographic groups may exclude them from job opportunities or perpetuate pay gaps.
It's important to note that bias is not always intentional or malicious. In many cases, bias is the result of unconscious assumptions or a lack of awareness about the impact of certain decisions. However, regardless of the cause, it's essential to address bias in deep learning models to ensure that they are fair and equitable for all users.
Real-World Examples of Systematic Oppression in AI
There are numerous examples of systematic oppression in AI. Here are a few examples:
Racial Bias in Facial Recognition Technology
Facial recognition technology has been found to be biased against people of color. A study by the National Institute of Standards and Technology found that many facial recognition algorithms are less accurate when it comes to identifying people with darker skin tones. This bias can have serious consequences, such as incorrect identifications by law enforcement.
Gender Bias in Natural Language Processing
Natural language processing (NLP) is the branch of AI that deals with language-based tasks, such as language translation or text generation. However, many NLP models have been found to be biased against women. For example, a language model trained on the internet may learn to associate certain occupations with a particular gender, perpetuating gender stereotypes.
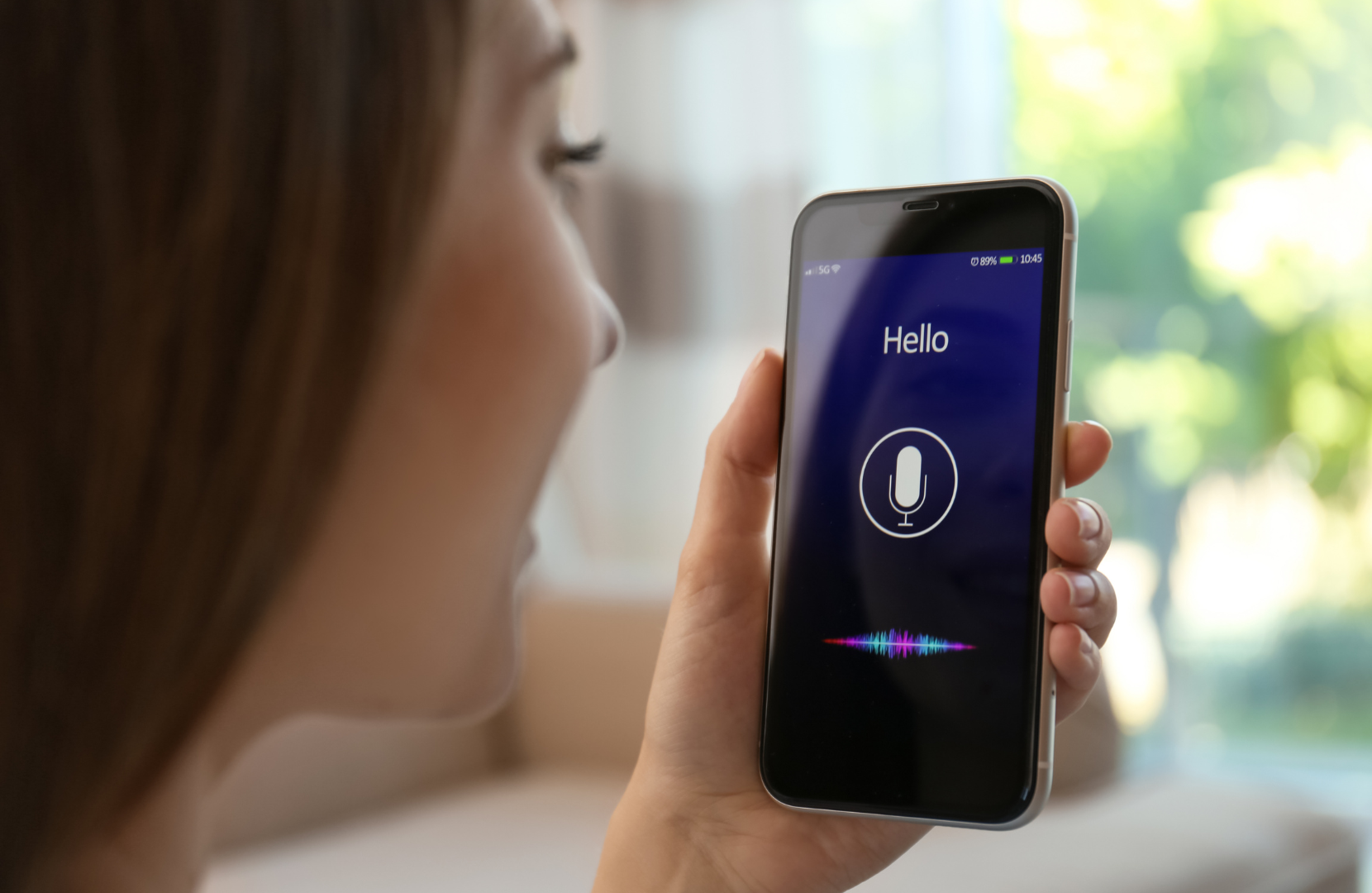
Socioeconomic Bias in Job Applicant Screening
Many companies use AI systems to screen job applicants, but these systems have been found to be biased against candidates from lower socioeconomic backgrounds. This is often because the data used to train the model is biased towards certain educational institutions or career paths.
The Consequences of Systematic Oppression in AI
The consequences of systematic oppression in AI are far-reaching. They can reinforce stereotypes, perpetuate inequality, and erode trust in AI systems.
Reinforcing Stereotypes and Inequality
When AI systems are biased, they can reinforce harmful stereotypes and perpetuate inequality. This can have serious consequences, such as incorrect identifications or exclusion from job opportunities.
Limiting Opportunities for Marginalized Groups
When AI systems are biased, they can limit opportunities for marginalized groups. This is especially problematic if these systems are used to make important decisions, such as admission to educational institutions or employment screening.
Eroding Trust in AI Systems
When AI systems are biased, they can erode trust in the technology. This can make it difficult to gain public support and may ultimately limit the potential benefits that AI can bring.
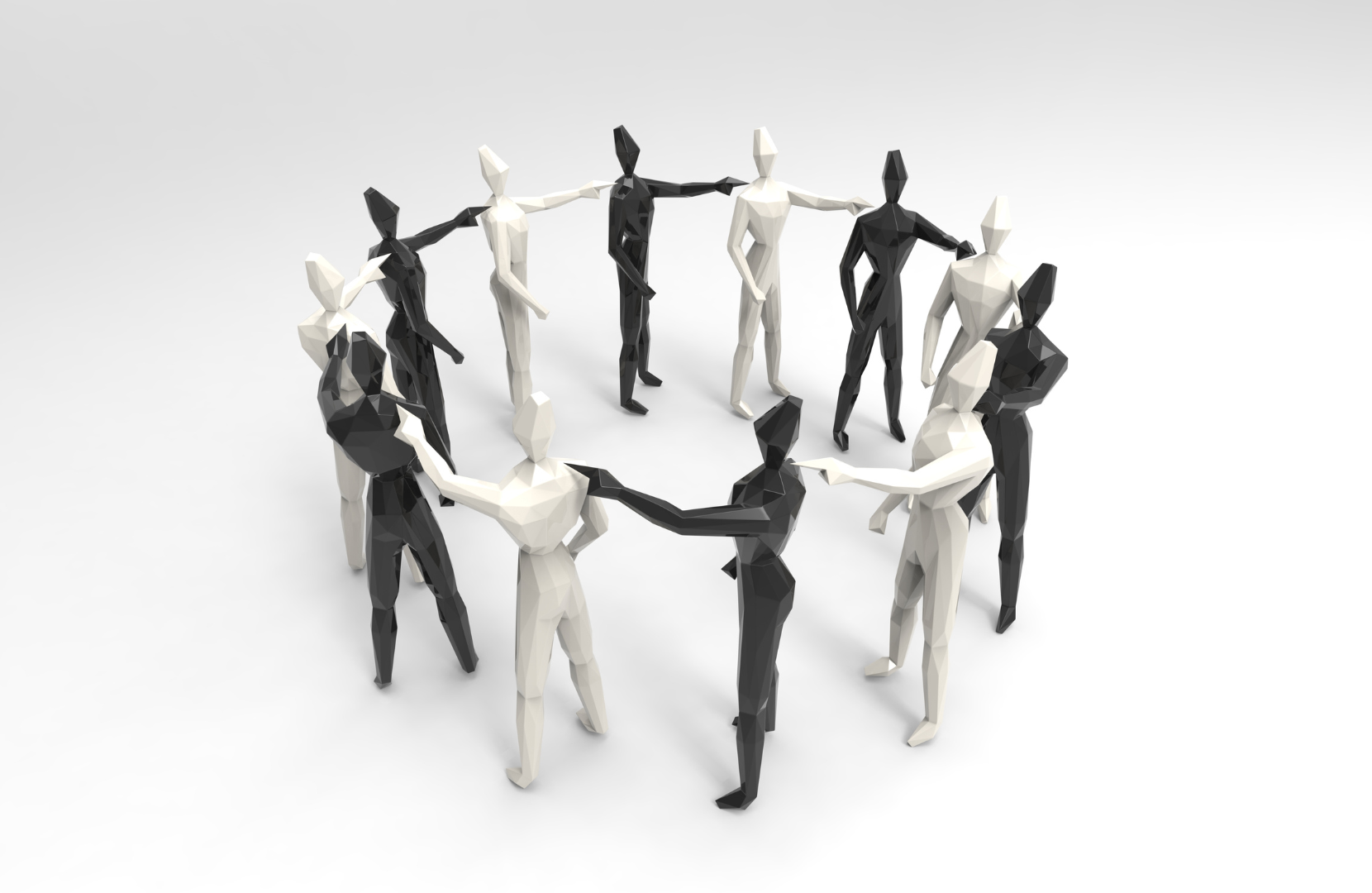
Strategies for Combating Bias and Oppression in Deep Learning Models
Despite the challenges, there are strategies that can be employed to mitigate bias and oppression in deep learning models.
Diversifying Training Data
To combat biased models, it’s important to use diverse training datasets. This can help to ensure that the model learns from a range of experiences and accurately represents all groups that it may encounter in the real world.
Implementing Bias-Mitigation Techniques
There are numerous techniques that can be used to mitigate bias in AI. For example, algorithmic techniques, such as debiasing and adversarial training, can be applied to improve the accuracy and fairness of models. It’s important to note, however, that these techniques are not perfect and should be used in conjunction with other strategies.
Encouraging Transparency and Accountability in AI Development
Finally, it’s important to encourage transparency and accountability in AI development. This can involve making the decision-making process clear and transparent, so that all stakeholders can understand how the system works.
Conclusion
Neutral deep learning models are a myth. They can be biased and perpetuate oppression, leading to serious consequences for marginalized groups. It’s important that we take steps to combat bias in AI, such as diversifying training data and implementing bias-mitigation techniques. Furthermore, we need to encourage transparency and accountability in AI development to ensure that these systems are used ethically and responsibly.